Biotech. What is it? Why should you care? Does biotech really matter for national security? What are China’s biotech ambitions?
To find out, ChinaTalk interviewed Jason Kelly, the Chair and Vice Chair of the National Security Commission on Emerging Biotechnology. Jason is the Co-Founder and CEO of Ginkgo Bioworks, a publicly traded firm that provides a horizontal platform for cell programming. Michelle Rozo is currently Vice President of Technical Capabilities at In-Q-Tel, and she previously held positions in Biden’s NSC, the Department of Defense, and on the Hill.
Co-hosting is Chris Miller, author of Chip War.
Here’s part one of our conversation, part two continues below. Or have a listen to the podcast! This was a really good one and the perfect onboarding point for this technology.
We get into:
Moore’s Law of synthetic biology;
Imminent pharmaceutical breakthroughs powered by the confluence of AI and genetic engineering;
Applications of biotech that can revolutionize military logistics;
The US vs Chinese innovation ecosystem for biotech
Where is Biotech’s ChatGPT Moment?
Chris Miller: Where are we right now in the development of ChatGPT-DNA?
Jason Kelley: Actually, this is a very important point for US strategy because we have not yet had our ChatGPT moment. We need to have ChatGPT for bio happen in the US before it happens somewhere else. The bones are there, it should work — but there are some missing pieces.
Do we have enough DNA code? If you think about the image models, it wasn’t just the image that it needed, it was also the labeling of the image. “Here’s a picture: it’s a dog.” “Here’s a picture: it’s a balloon.” In the biotech case, we need, “Here’s a gene, it does … what?”
We need to generate the datasets that do the labeling of what all that DNA does. We need tons of that. The key question is going to be, “Who builds the datasets first?”
Chris Miller: How will we know when we’ve gotten ChatGPT for DNA?
Jason Kelly: I don’t know. I remember that, before the GPT moment, we were kind of wondering what the indicators of an AI breakthrough would be. “Maybe robots will be folding laundry, maybe AI will be playing chess, maybe it’ll be designing soda flavors” — we didn’t know!
There have been little hints. Famously, Google created a product called AlphaFold. Remember AlphaGo, for playing Go? They made AlphaFold for modeling the structures of protein folding. This is the nerd problem in biology — if I give you a sequence of DNA, what protein shape does it make?
Proteins are the little pieces of nanotechnology inside cells that do things. Science has been trying to predict those shapes forever and failing, failing, failing. They run a competition every year, where somebody secretly figures out what the protein shape is in the lab, doesn’t tell anybody, and has all these computational people try to predict it.
And then the winner is whoever predicts the most of the fifty proteins that year. In 2018, Google’s AlphaFold entered the competition and finished middle of the pack. In 2020, they basically ended the competition.
So we’ve seen little bits of potential, but not a ChatGPT moment.
Michelle Rozo: I have two answers for you, Chris. One metric that we could look for to see that we’ve reached the ChatGPT moment in biology is actually getting the outcome we want with the products that are being designed through AI.
An example of this: most of the drugs that go through clinical trials today fail. Nearly 95% failure of the drugs that we put in a clinical trial will not succeed. They will not actually make it to patients. AI tools have started designing new drugs, with new targets, using new types of molecular interactions. They’re uncovering interesting stuff, but AI has not yet had the effect we’ve wanted to see on the drug market.
Now perhaps those drugs have a pass through clinical trials at a rate of maybe 6% to 8%. When we start seeing that number go way up to 70% or 80%, when we see much more efficacious drugs with targets that are clearly defined — and in clinical trials that are designed through the use of artificial intelligence tools, we will see the output in terms of more numbers of successful drugs coming to market and coming out of clinical trials.
A second thing that we need to look at, though: we may not know when we’ve hit the GPT moment in biology in the same way that we did for ChatGPT, because by and large, these tools and these applications are being designed by independent private companies. These are proprietary tools and proprietary datasets. Unlike ChatGPT, where we had this function that was released to the world so that you could see the capabilities for yourself, biology may not have that same moment. These tools may not be accessible to the broader public. We may reach that moment inside individual companies, inside individual countries, but perhaps we may not understand from a broader societal perspective that we’ve actually reached that moment.
Even though we talked about how we don’t even know enough about biology — biology itself has tried only so many combinations of potential protein sequences. So the ability to design things de novo, anew, things we’ve never seen before with new functions — that will be an important potential indicator.
These delayed outputs — increased numbers of products making it to market, or novel designs of things that we’ve never seen before in nature — are probably going to be the best indicators. Once those things start actually hitting mainstream product markets, then we can probably say that we’ve reached that moment. But again, that will be a delayed delivery.
Jordan Schneider: The idea of going from 8% to 50% is crazy. You basically have to have AI predictive models of an entire biological system — and not just a microbe either, but a whole human or a monkey or a horse.
Jason Kelly: What we’re learning with the human language model is that we don’t need a perfect model of a system. We’re not trying to do physical simulation with neural nets. We’re trying to do extreme pattern-matching.
The way you’ll do this, for example, would be to collect huge amounts of data about human cells in the lab. You need data about how different types of things affect human cells — and by “different types of things,” let’s say proteins for example.
Then you’re going to use that data to train a model to design, for example, a protein with a shape such that it has the desired curative property but doesn’t set off the human immune system.
Overwhelming immune response is one very common reason that a cancer therapy might fail. If the immune response hurts the patient more than it helps, then that’s a failed clinical trial. So we’re looking to design these proteins such that we can avoid that ever happening.
Well, collect enough data about what immune responses you see in response to a wide range of things, and the neural net will pull the pattern. We might not actually have a model that encompasses the whole human immune system, but it doesn’t matter. As long as the neural net has seen enough coherent “sentences” written with the correct “grammar” to not set off your immune system, then it will be able to write sentences of its own — just like ChatGPT can write in the style of Shakespeare, even though it doesn’t have a model of Shakespeare’s head.
Michelle Rozo: There are other models too that are not based on this type of fundamental DNA language that Jason’s talking about. You can design AI models to go through health data, health records, and clinical trial information to actually design the trial better. You can train it to pull in the right populations to gain understanding of the targets you wanna achieve. It’s not just the drug itself, but every application of making the drug — down to the robotics involved in manufacturing — each of those levels will involve artificial intelligence.
It will be different types of different models, but the entire pathway can stand to be accelerated — getting a drug from design, getting it into someone’s arm in a clinical trial, getting it approved by the FDA and then to market — by different combinations of artificial intelligence tools and datasets.
Moore’s Law of synthetic biology
Jason Kelly: These fundamental abilities of biology are so powerful that even bad tools of the 1970s, 1980s, and 1990s were valuable. In the 2000s, you had genomics to help you do the reading. Then in the 2010s and 2020s, we entered the era of what people have been calling “synthetic biology.” The tools underpinning this field kind of mirror the tools of the Genentech era.
The first tool is DNA synthesis. So, instead of cutting and pasting, I can now go on my computer, type “ATCGGGGGG,” hit print, and a California-based company called Twist Bioscience will print the piece of DNA I want. I don’t need to cut and paste anymore; I can just type in what I want. It takes some time to get it, depending on the size, but they will ship me a tube in the mail that contains my custom DNA code.
That was a major breakthrough, but you still pay by the letter. For the computer scientists, I want you to imagine that as you compile your code, each zero and one costs you a few cents. That’s a major limitation.
Second, the restriction enzymes that Genentech had? The scissors? The new ones are called CRISPR. Restriction enzymes would only cut in the same place every time. With CRISPR, you can choose where you want to cut. Importantly, it works not just in microbes, but also in plants, animals, and humans — which is a huge breakthrough. Before CRISPR, Monsanto was just shooting DNA randomly into the corn, hoping it didn’t mess something up, and picking the winners. With CRISPR, you can pick where you put it, so it’s way more efficient. You get way more success in terms of not messing up what’s already there, because you can put it somewhere purposefully.
The third technology for synthetic biology is genomics. We collect code data and we do high-throughput automation to test what all those genomes do. That gives us a genotype, which is the DNA code, and a phenotype, which is laboratory automation-produced data about what that genotype does in the world. It’s a giant corpus of many, many more operons.
The tools of synthetic biology are DNA synthesis, CRISPR to insert it, and then high-throughput biology research, which is a mix of genomics and automation so that we can learn biology faster than ever before. You put those three things together and you’re in the synthetic biology era, which is the one we’re in today. I personally think that this is the one that has compounding gains.
It was planar semiconductor manufacturing that created the fundamental pacing of Moore’s Law, and you just knew it was going to get better and better and better. We are now starting to see that in synthetic biology.
Michelle Rozo: We’re still on the inflection curve. They’re getting better all the time, but there are gaps that still exist in that corpus of knowledge. We just don’t have enough genomic information yet.
On the DNA synthesis side, we’re good at writing short amounts of code. But if you want to get longer and longer, that’s technically challenging, and we haven’t solved that yet.
Those three technologies that Jason mentioned are still in rapid phases of acceleration. We’re getting better at this all the time. New companies are forming around each of these intersections. As he highlighted, it’s increasingly converging with artificial intelligence. The industry is still on an upward trend.
Jordan Schneider: We’ve had a nice fifty-year run. We went from zero to a trillion-dollar industry. We got some great plant yields, we did some mRNA vaccines, saved the world from COVID, and all that good stuff — but you two are saying that the best is yet to come. What innovations are you two looking forward to the most?
Jason Kelly: The curves that I’m most excited about are DNA reading and DNA writing. These are both getting cheaper in a way that kind of aligns with Moore’s Law.
I’m also excited about laboratory automation to generate data about how cells work. Again, we didn’t design cells, so unless we actually measure them a lot, we don’t really know how they work.
Then the last innovation I’ll mention is the generative AI models you build on top of that big data asset. You are starting to see cloud labs and business models where that’s outsourced, like in AWS.
Michelle Rozo: I think the basis of what Jason’s talking about is we need to understand biology better. We then need to be more efficient in how we do that engineering that he’s so nicely walked through. Then we need to do it at scale. Reproducibility in science is difficult, and because biology is living, it’s inherently challenging.
That insulin example sounds kind of simple in retrospect, but actually to get that done at full scale and make enough human insulin via a bacteria that can be used on our market was a massive undertaking that we shouldn’t under-appreciate.
One area that the field is kind of by and large struggling is to move these basic lab innovations out of the lab into and into large-scale manufacturing. That comes back to, again, how much we know about the biology, how much we can engineer it. As we start to pull all of these things closer together, and do the engineering with manufacturability in mind, we start to transverse that curve from lab to market much quicker, which brings a lot more of these potential products and applications that we’ve been talking about to the general consumer.
Chris Miller: We mentioned Moore’s Law, and the rate of declining cost per transistor is historically unparalleled, I think. But I understand there are some technologies in the biotech sphere that have had roughly comparable cost declines over the past couple of decades. Where do you see the cost trend going?
Jason Kelly: This is a very good question. The example that gets talked about the most is genomics. The cost of DNA reading has fallen faster than Moore’s Law over the last twenty years, so it has gotten dramatically cheaper. The Human Genome Project required roughly $100 million to sequence one genome. Today, companies like Illumina and Beijing Genomics are both orbiting around about a hundred dollars per human genome. So we’ve seen a 1 million-fold cost reduction in the last twenty-four years. That’s legit even to the semiconductor people.
By the way, interestingly, the technology leans on semiconductors, but that’s a little far out into the weeds.
Jordan Schneider: No no, please elaborate.
Jason Kelly: The technology that Illumina is using to do this low-cost sequencing is leaning on historical innovations in semiconductor manufacturing to make portions of their chips. It’s essentially a really high content-imaging application to get this right.
You’re looking at billions of little spots that are each little pieces of the DNA. The process of fabbing that thing is very much driven out of progress in semiconductor manufacturing. It’s an overlapping technology, but a completely different use case. The lithography is 100% piggybacked on progress in chips.
Then of course the other technology that is getting less expensive is DNA writing. It’s not gotten as cheap as quickly as DNA reading, that’s for sure. That’s an area where more investment would really serve as an unlock.
Think of yourself as a computer programmer paying by the bit: when you compile your code, you would learn a lot slower. Your development cycles would be more expensive. That’s a real gate on the industry.
Imagine programming for a computer that you didn’t know the computer architecture for, and for which no one had ever built an operating system that simplified it.
We’re kind of installing assembly code — or even bit code — straight into a machine that is not of our design. There’s a whole world of improvement that is just on the knowledge side that Michelle was highlighting.
That’s where I think AI models are going to prove to be extremely important, and in fact, they are already showing breakthroughs.
For example, in the design of proteins, AI models are now the best in the business. That is a new development in the last five years or so.
Michelle Rozo: I do think it’s important at this stage to stop and highlight where we’ve been and where the field needs to go. Where we’ve been has historically been good matches for government funding. We’ve heard how government funding has supported that kind of basic R&D. We had this great challenge around the Human Genome Project. That sort of fundamental research is a function that the US government does well and has supported kind of the basis of this industry. But as we have a field that needs to undergo some continual improvement in some of those technologies, which involves more directed types of human-genome-equivalent type national projects, as well as a transition.
We’re really trying to figure out how to get out of lab for some of these capabilities and into the marketplace — and particularly for some of the defense applications of these technologies, which we haven’t talked about yet. But we can go into more detail of how the commercial market applications, and then how the Defense Department may want to use some of the innovations around self-healing and materials and responsive materials.
They’re going to look different. And so that market shift, that strategic lens, and the transitioning of technology is something that the US government has historically been poor at funding. That’s not the function that the government has traditionally taken. Of course, that’s changed with investments in chips and science and some of the recent laws that have been passed around investing in infrastructure.
We’re starting to see the government use different tools around tech transition and fundamental types — non-fundamental types, I should say — of investments, but that is an interplay with biotech where you’re getting to a stage of development in the field that doesn’t complement the historic US government tools and means of funding.
The US innovation ecosystem and competition with China
Jordan Schneider: We have a potential future unlocked where we have robots in labs doing tests, AI helping to simulate drug applications — and all of a sudden we go from what’s today a trillion-dollar, market to ten trillion dollars, and we get to grow the best-tasting, most efficient corn you’ve ever had, live forever, and so forth.
But what role does the government have to play in achieving that future? Looking at the AI analogy: if you pulled the plug on NSF computer science funding in 2010, you probably still would have had enough infrastructure and support available in corporate R&D to unlock the ChatGPT moment seven to ten years later.
Where are we in the life cycle of our supernova of biotech capabilities? What you can expect VCs and CVCs to develop, and where will the government still need to be involved to unlock this glorious future?
Jason Kelly: I don’t have a great answer to the bulk of your question, but I’ll tell you my favorite future application for advanced biotech: everyone always thinks about drugs and the things we can do with biology today, but I’m actually most looking forward to advancements in chip manufacturing and semiconductors. Biology places atoms really well.
Michelle Rozo: It’s clear that the pharmaceutical applications will continue to be developed, because there is a market for high-performance drugs. We’re seeing more and more types of drugs enter the market all the time. We have new weight-loss drugs like Ozempic which target the GLP-1 receptor. These drugs have the ability to not reach just a specific defined clinical population — say, someone that’s suffering from heart disease or cancer — but actually large percentages of our population. We’ll see more and more of those types of drugs that have larger and larger market sizes. The market will always, I think, continue to bear out some of those advances.
In my view, the other applications of advanced biotech will struggle without additional government support or allocation of strategic resources. Jason mentioned some of the agricultural tech examples. There are large challenges in placing genes in plants versus in bacteria.
There’s still some fundamental research needed in order to improve our understanding of plants and how to engineer them. It’s much more challenging than doing it in microbial cells. If we look toward agriculture applications, there’s a larger role for non-dilutive sorts of funding and for government funding.
The industrial sector is a completely different story. There’s the potential for biotech to make products that displace some of the industry incumbents. You can look at replacing petrochemicals with biologically produced sources of those chemicals, but the market doesn’t support that today. Large petroleum companies and entire industries were historically founded around the use of petrochemicals to make plastics materials form the basis of our supply chain.
While there may be strategic reasons or environmental reasons to diversify away from the traditional petrochemical basis of our supply chains, and use biotechnology and biomanufacturing to make those products instead — that isn’t going to happen without additional support from governments. We’re seeing how different governments are tackling this in different ways on the international stage. In Europe, for example, they have specific penalties or “sticks” around carbon emissions, so there’s more of a push to develop biotech in order to accomplish climate-policy goals in the EU.
There are areas where the market will continue to drive biotech, and then there are areas where there is no market today.
Jordan Schneider: All right. So we have some R&D gaps. Reading your report, it sounded like it’s a mess in terms of who is in charge of this. It seems like it’s basically you two thinking strategically about this without a cohesive institutional home in the government.
What are the different constituencies that should care about biotech? Why haven’t we planned out a cute little integrated biotech strategy to push this forward over the next twenty years?
Michelle Rozo: There are plenty of smart government people thinking about biotech, but in one way this is a symptom of biotech. But it’s also a diagnostic of our entire government as a whole.
The government has not historically been able to support convergent technologies. As we’ve heard, biotech is inherently a convergent technology as it accelerates. It’s not just biotech: it’s biotech with AI and with automation. There are crossovers with the semiconductor industry, both because semiconductors need to be used in the underlying equipment, and then Jason will tell you the story of how biotech can transform semiconductor manufacturing in the future.
There’s potentially a quantum aspect in computing. There is this grand convergence of technologies and our government just doesn’t effectively build policies for that. We are siloed. We have different agencies with different priorities. Each agency has its mission. They have swim lanes, and they are moving biotech along within the confines and in the direction of their swim lane, and they’re doing it by and large well. There are great programs, great scientists, and we’re seeing the translation of very early R&D into applied R&D. But asking the government to function in the capacity of looking beyond those defined mission areas and swim lanes is just not something that the USG has historically done or done well. So biotech can be used as an exemplar for emerging technologies as a whole. All of our technologies are increasingly converging.
We know that we struggle as a government with moving transitioning technologies from the lab and into the market. We need to look at how we can apply new tools, where we might need additional tools, and how we can break down some of the silos — this is true regardless of what technology you’re talking about. That’s what we need in order to arrive at some of the outcomes that we all can agree would be great for the United States.
Jason Kelly: What do you think is the origin of this difficulty with convergent tech?
Michelle Rozo: That’s a good question. It fundamentally comes down to the way that we’re trained in these technologies. It’s already challenging to get scientists into the government, and those who are there were trained a while ago. When both Jason and I were trained, there was a strong focus on single-disciplinary training.
Now we’re moving into these multidisciplinary programs. But those people who can speak AI and biology and semiconductors all at the same time are still unicorns. They are widely needed and desirable in the technology innovation front, and they can recoup massive salaries there. Getting those people in the government is one hurdle that limits the understanding of our policymakers.
Another issue is just the mission space of these agencies. Besides the Commerce Department, which has a broad commerce lens, our other agencies are limited and focused in scope. The Energy Department does energy. Our Health Department does health. Our Defense Department can kind of fit all of those in, but of course, through the lens of the military. When we think about biotech being applied, it’s done so under those domains. We’re creating artificial boundaries between biotech for health and energy and defense. If our system was structured differently, we could apply biotech R&D priorities that could advance all of those missions, not individually, but rather in a parallel or in a concerted fashion.
Jordan Schneider: It’s interesting to think about the comparison to the AI executive order, where you had literally every department and government agency coming up with their own strategy. The degree of difficulty is even higher for things that are not priority #1 for the government.
Biotech is a secondary thing where we’re really excited about what it’s going to do for us in 2027 or 2030. But post-ChatGPT, you have the president talking about this all the time. Unless the White House is screaming about the issue, it’s difficult to force the government to develop things horizontally. It’s structurally tricky for the US government to wrap its head around.
Michelle Rozo: It’s even more concerning when you apply the lens of competition with China. We don’t look at these things in a cross-cutting way, and their system does. They’re inherently more risk-tolerant. They can fund things from a top-down perspective in a way that is not encumbered by the government system. Of course, there are limitations in their system as well. But their ability to systematically look at emerging tech (in a way that our system has struggled) is something we should be concerned about. If we are going to outcompete in all of these domains, we need to do something to address those inefficiencies in our system.
Chris Miller: I have a question about the economics of all this. The government is funding basic research, and we’ve got capital markets that, at least from my perspective, are putting a lot of money into biotech firms. Where are their funding deficits right now? Is the issue with scaling up capital-intensive parts of the business?
Jason Kelly: On the emerging biotech side, we need new tooling. The analogy with AI goes something like this:
There is a nonprofit called Common Crawl, which is a big internet text database that basically all the foundational AI models used to train their models for human language.
Then there was ImageNet, which was an open-source labeled image database to use in training AI models. There were these big public sets that were available to researchers to just work on, to try to work on the problem of AI in the domain of images and human language.
We need those in biology. In biotech right now, there’s a lot more custom proprietary stuff.
We have little bits and pieces of databases, such as the Protein Data Bank which AlphaFold was trained on, but those are few and far between. The government could lead in the creation of some of those shared public assets. That would then allow open-ended research to happen in the beautiful way it does in the United States, where people just do it permissionless.
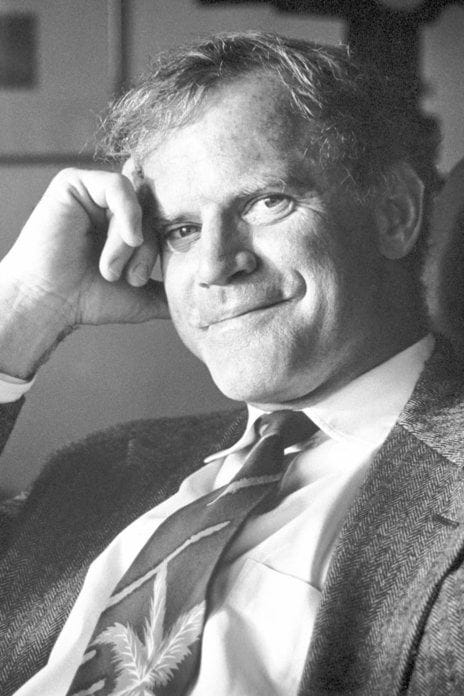
They don’t have to be blessed by the government — they can just go to town — but we need those core assets. With the right little bit of push, we can create an enormous amount of innovation downstream in our system. No VC would fund the creation of ImageNet. It’s a systems-level benefit. It’s not a benefit to that company. It wouldn’t pay to create all that data.
Michelle Rozo: It’s also the application areas that are ill-suited for VC funding. Most of the VC funding that you see in biotech is for biomedical.
Firms that are trying to orient around non-medical applications are really struggling to receive financing. The time horizons are the same with ag biotech and industrial biotech, but the potential return is less than for pharmaceutical.
There are also fewer success stories. We’re not seeing as much of this novel biotech yet being applied in these sectors simply because the margins aren’t the same as in the pharmaceutical sector.
Jordan Schneider: Michelle, can you make the pitch for combining biotech with national security?
Michelle Rozo: We’ve talked about the economic potential and the ability to solve for big strategic goals within the United States — like taking entire diseases off the table, solving food insecurity, and addressing environmental challenges.
We can apply some of the same technologies for specific priorities that will increase the national security of the United States. As we saw during COVID, our supply chains are increasingly fragile. We depend on foreign sources for a lot of the inputs to our economy. This creates not only economic challenges but also national security challenges. Biotech can help us there. We’ve talked about the potential to use biomanufacturing as a way to source critical chemicals.
An example is active pharmaceutical ingredients. This is a core component of our drugs. The supply chain around these is murky. We don’t actually know where a lot of these core components are coming from, but it’s clear that the majority are not coming from the United States. That puts our population at risk in times of crisis, if we cannot get and secure the necessary ingredients to make our drugs and treat our population. A lot of these drugs are being imported through China. Again, how much is still unclear, but it is clear that they are an important node in that supply chain.
These active pharmaceutical ingredients are either sourced from nature, or they can be made through chemical synthesis. This type of chemical manufacturing is a dirty way to make these products. It creates a lot of toxic runoff. It’s also highly energy-intensive, so it’s not the best working environment. You can do it at a really low cost, but these types of manufacturing were offshored from the United States. We don’t necessarily want this type of manufacturing in our backyard anymore.
However, we could instead make new means of production for these active pharmaceutical ingredients using biomanufacturing. Creating cell factories that instead are engineered to produce those chemicals through their metabolic pathways would be environmentally friendly. They would not create the toxic outputs that motivated the initial offshoring.
Our Department of Defense also relies on foreign sources for critical components of its supply chain in the same way that our broad economy does. Biomanufacturing could be used as drop-in replacements for those products.
It also can create new enhanced capabilities and enhanced performance. You can think about the size and the ability to scale manufacturing with a brewing beer analogy. Full-scale manufacturing happens at a national company, but you can also scale down to your local brewery and even down to a home brewery. That’s scalable. It’s also more portable. You can do it on demand. You can do it where you want it.
If you think about logistics — the ability to resupply troops on-demand with fuels, lubricants, energetic precursors, and medicines right in the theaters in which they need them would be hugely valuable. Conventional military supply chains are time-intensive and potentially disruptible. There’s a massive capability unlocked if we can do that at scale.
Imagine — materials that can sense and respond to a potential weapon, materials that can identify if a soldier has been impacted by a weapon, fuels that provide long-range munition power because they’re higher energy density, temperature coatings that can withstand hypersonic flight. We’re just scratching the surface of what is possible, because all of that basic research and what we understand about biology is even slower to translate to the Defense Department.
The more we understand about biology and the mission space of the Defense Department, the more we can increasingly integrate those. It was only in 2018 that the Department of Defense announced biotech as a technology priority area.
Jordan Schneider: Michelle, that's cool but I want to jump 10 feet tall and live forever and have bullet wounds heal themselves. Where are we on that timeline? How is the department thinking about potentially taking advantage of the sci fi stuff?
Michelle Rozo: Yes, there are some more far future applications of biotechnology that could really transform the military environment and operating environment.
And some of these are really exciting potential to keep our warfighters safe or heal them in the fields. But we're really at the beginning stage of some of this exploration. So I like to break it apart into a couple of different domains where biotech is going to have a capability potential for the department.
One is just on broad military capabilities. Your stuff, your hardware. Think: tanks, armor. The things that equip and serve our military service members, but not them themselves. And so we've talked about how biotech can provide drop in replacements for the materials and capabilities and products that the defense department uses today, but we're also seeing the potential for enhanced capabilities in those products. So materials that are responsive potentially, that are able to detect something in the environment and respond accordingly and impact back to the service member. These are still all research stage, but it's really exciting potential. The next domain is human performance, and so from the Department of Defense, a lot of this is around training.
How do we design the right atmosphere with which to deploy our soldiers, understand where, how to train different people for different environments. But there's also some interesting basic research around microbiome treatments, for example. So giving someone like a probiotic that could respond to a disease to what's going on in their body. And let's say they need, they're tired. They need a kick of energy being able to deliver that and respond to someone's performance in real time. Those are, again, early stage, basic lab types of capabilities, but things that are interesting to think about. And then probably more traditional applications that one thinks about when they think about biotech and then Department of Defense are around military medicine.
How do we heal someone? This could be something like regenerative medicine, new tissues, new, synthetic blood, potentially even, entirely new organs or, features like that, which are, again, still, still far out there, but really exciting to think about. And then medicine at the point of need.
So being able to make some of those biological products where they're needed, where someone may be injured or hurt. And then finally, chem biodefense. And so how do we respond to the threat that may be provided by biology by someone misusing that or chemical weapons, and being able to employ biotech as a solution space.
So all of those are under exploration at the Department of Defense. As I've said multiple times, these are nascent, depending on what you're talking about, but really there's a lot of cool proof of concepts, lab research, partnerships with academia and with companies that are developing some of the starting materials for some really cool capabilities that can impact everything from the systems that our warfighters use to what they're wearing to how they can be fed or healed, as needed and where needed.
Jordan Schneider: The perfect book to give to a 21 year old just starting to get into Nazis, this book called Blitzed Drugs in the Third Reich, which basically makes this argument that every Nazi soldier was up for 20 hours a day because they were all on speed. And that was fine for year one and year two of World War II, doesn’t end up great by 1944…
People have been drinking coffee before going into battle for millennia now. Apparently, all the Navy SEALS are on steroids.
For a lot of these different things is it's not just military applications where people are going to be excited to take a pill and get smarter, or be able to sleep less, or be able to heal a wound from a car accident or what have you.
What is your sense of the role of what defense-oriented funding needs to be doing for this versus all the stuff that Jason talked about earlier, where once we hit these exponentials that are going to have enormous commercial outcomes that will sort of end up flowing down into these military ones as well?
Michelle Rozo: You raise a great point where I think a lot of the actual acceleration of this technology is going to be in the commercial space, and not even in the medical space, but in sort of the performance type of less regulated kind of arena, right? Like that's beyond FDA. If you want to take a caffeine supplement, that's not prescribed, right?
We haven't even talked about brain computer interfaces and some of those potential applications. But I think this is where the role of the government comes in, right? And where the Department of Defense kind of seeding a lot of basic research capabilities, but then a lot of that goes into the commercial or academic world and will be continued to be developed by industry and outside of government.
Jordan Schneider: Speaking of China, why should I be worried if China has my DNA?
Michelle Rozo: The genetic information is a good one. I think we talked about earlier about how genetic information and sort of the code base is going to be one of the underlying elements that is driving the future of biotechnology, right? And whoever has the largest code base or the most diverse code base in this case has a chance to win out.
We're looking at the connection of AI and biotech. That's very important from a competitive angle. From an individual level, your genetic code is specific to you. It's identifying as a person, and there are some inherent risks to someone being able to identify you as a person, just from an individual level.
If you think about blackmail or other sorts of types of targeting of an individual. You and I might not be worthwhile of being targeted by a nation state,
Jordan Schneider: Hey, speak for yourself…
Michelle Rozo: Ha!
Then from sort of a macro level, we talk about all of the potential for good and commercial success out of building out a large code base for biology and being able to design products against it.
But we must also be mindful that that same sort of information could spur the development of biological weapons that could evade detection, evade our ability to respond to them, and it's something that we're mindful of, of sort of this unknown potential that would be damaging and detrimental to our system if developed and utilized.
Jordan Schneider: Can we make a drug that only kills Jordan Schneider based on my DNA?
Michelle Rozo: I think it's theoretically possible. We're still at the early stages of being able to understand that, but it's clear that AI and bio is being applied together at the very beginning stages of being able to design new biology. I think we're at the level of small molecules, it's working somewhat well.
We talked about this in the drug space. Proteins were at the beginning stages of that. Getting to the level of a virus and a higher order organism, we're still away from that. But I think the potential consequence of this and what capabilities that that intersection could enable from a weaponization perspective is still unknown and therefore something we need to be mindful of.
A call from Uncle Sam
Jason Kelly: For the folks reading this at home: the whole point of the National Security Commission on Emerging Biotech is to collect input from the community and then turn that into information for the government. If you have insight about the interface between emerging technology and national security, please do reach out.
I’ve realized that the government community is so independent from the private sector and even from academia. It feels as though these are just very different worlds — in part just because people don’t feel it’s their place to engage. Engagement between these domains has certainly been very valuable for me as a US citizen.
There are great people in both domains, but there’s so little crossover. It’s a weakness honestly in our system as a whole, and I don’t know the right solution to that.
The private sector thinks about interacting with the government as some kind of regulatory trench war. But from the lens of competition with other states, government and industry need each other. We need to be better at crossing that divide.
Michelle Rozo: Then on the reverse, Jason, your company has been successful in hiring former government employees, right? That’s also something that’s lacking — is the ability for government officials to actually understand what’s happening in the private sector, especially the financial realities.
The government needs to know where the tech actually is and the state of the intersection between public and private capital. The folks who are making policies about this are often too removed from the companies that we’re trying to promote or protect.
Jordan Schneider: Is this all happening because Newt Gingrich killed the Office of Tech Assessment twenty years ago? Or do you think there’s something more structural going on?
Michelle Rozo: I think it’s the latter. The tech is not happening inside the government anymore. Government funding for technology has declined. The private-sector scientists and companies are at the leading edge of the technology and therefore understand better than anyone else. I think it’s too much to expect the government to be able to do that in addition to its functions. We need to start with realistic opportunities for collaboration.
Jason Kelly: Maybe I’m just optimistic because I’m an outsider, but back in the 1940s and 1950s, obviously government was doing big technological projects all the way through the Apollo program.
Remember, OpenAI was a non-profit when it started. If you had just given Sam Altman the budget to do OpenAI in the government, he would have done it. This idea that the government can’t recruit amazing people to do amazing technological things is not true. They just think they can’t. If given the budget to recruit out of the private sector, you could get Elon to run NASA. I swear to God, this would work.
You’ve got the checkbook, man. The budgets are bananas. The government doesn’t think it can do capital allocation like that anymore, but it certainly used to: see the Manhattan Project.
The other thing they get in the private sector is control — they get to spend that money the way they want to spend it.