With DeepSeek’s recent breakthroughs dominating headlines, a deeper question remains: is the Chinese government truly pushing toward AGI? And if so, what role if any might it have in allocating compute?
While DeepSeek’s accomplishment suggests that access to compute beyond what a hedge fund can cobble together may not be an immediate bottleneck for developing algorithmic advances, the picture is more complex. Western labs are still racing to build massive 100,000-GPU-scale clusters (not to mention the Stargate Project) in the hopes of developing and deploying AGI at scale, and DeepSeek’s CEO has repeatedly said that he is compute constrained.
In this article, we unpack a key paradox: how can China have simultaneous compute overcapacity and shortages? And what does this say about whether China is ready for the next step towards developing and deploying AGI?
Since early 2024, reports have emerged of idle computing centers in China. And in September, renting Nvidia AI chips in the cloud was cheaper in China than in the US.
At first glance, this doesn’t make sense. Since the US restricted China’s access to advanced AI chips, one would expect to see chip shortages, every available chip used to its fullest, and high cloud prices.
So, what’s going on here?
The puzzle has also attracted the attention of Chinese journalists. In this post, we synthesize takes from two long Chinese articles from November of last year.
China’s Computing Power — Is There Overcapacity? 中国算力,过剩了吗?, written by Wu Junyu 吴俊宇 for Caijing Magazine 财经杂志. (link, archived link);
Too Many AI Computing Centers, Yet Not Enough Large Models 智算中心太“多”,大模型不够用了, written by Zhang Shuai 张帅 for TMTPost 钛媒体 (link, archived link).
Key takeaways
China has added at least 1 million AI chips (70% Nvidia, 30% Huawei) in 2024 to its compute capacity — enough to build, in theory, several 100,000-GPU-scale clusters (which is what would likely be needed to compete with GPT-5 or other next-gen Western models).
But how come even in the current 10,000-GPU-scale cluster era China already sees overcapacity?
Explanation 1: chips are poorly deployed, leading to shortages for “high-quality compute” but overcapacity for “low-quality compute.”
During the AI boom, many companies and local governments rushed to buy GPUs, assuming their mere ownership would ensure profits.
Since many of them lacked technical and market expertise, however, chips ended up in geographically dispersed, low-quality data centers that are either difficult to use or in places without demand. This speculative behavior and resource mismanagement have given rise to idle computing centers despite overall shortages.
The government has responded by no longer granting permits for new data-center construction, unless they are located in one of eight designated hubs. Industry consolidation and professionalization make it less likely that the same kind of chaos will hamper China’s data center construction in 2025.
Explanation 2: short-term overcapacity, long-term shortages.
By 2024, the demand for foundation-model training has significantly slowed. Many of the players that rushed to develop foundation models in 2023 have ceased training new generations.
Although inference demand is growing rapidly, it remains insufficient to fully absorb the excess capacity previously dedicated to training.
In the future, rising inference demand and the development of larger foundation models are expected to drive new shortages. The current “transition period” of temporary overcapacity is anticipated to end in mid-2025.
Below, we go into more detail on what the Chinese media has to say on
How many AI chips China has, and who is deploying them;
The emergence of “fake” and “pseudo” 10,000-GPU clusters, and how the government is responding;
Unique challenges for compute clusters using Huawei chips;
The transition from training to inference demand;
Whether China needs more public cloud computing and fewer private GPU clusters;
How China can avoid pitfalls from the 10,000-GPU-scale era in the 100,000-GPU-scale era.
Who are the major players investing in AI computing centers?
To start with, Caijing gives an overview of who actually builds compute clusters in China. There are three major groups:
large technology companies (Alibaba, Tencent, Baidu): projected combined 2024 capex of over 130 billion yuan;
state-owned telecom operators (China Mobile, China Telecom): planned 2024 investment of 84.5 billion yuan;
local governments and SOEs: statistics are very incomplete, but at least 27.5 billion yuan investment from January to October 2024.
Caijing goes in depth on the data to estimate the total number of chips put into operation by these three groups in 2024.
Large technology companies
Caijing argues that capital expenditure is a good proxy for computing-center investments, since capex is usually used to purchase chips and lease land.
In 2024 H1, the total capital expenditures of Alibaba, Tencent, and Baidu reached 50.44 billion yuan, up 121.6% y-o-y. This is the highest growth rate since 2018. If the growth trend in the first half of the year continues, the total capital expenditure of Alibaba, Tencent, and Baidu will exceed 130 billion yuan in 2024.
Note that Huawei and ByteDance also operate major computing centers. But because they are not listed companies, they have not disclosed relevant data — which means the total investment in the “large tech companies” category is actually higher than the data presented here.
Expect DeepSeek in the coming months to shack up with one of these firms just like OpenAI did with Microsoft. TMT 钛媒体 reported on Monday that ByteDance is “considering research collaboration” with DeepSeek. Partnering with ByteDance, however, could be an enormous unlock for DeepSeek researchers, giving them access to orders of magnitude more compute.
Figure 1: Capital expenditures of Alibaba, Tencent, and Baidu (Q1 2021 – Q2 2024) Unit: 100 million yuan
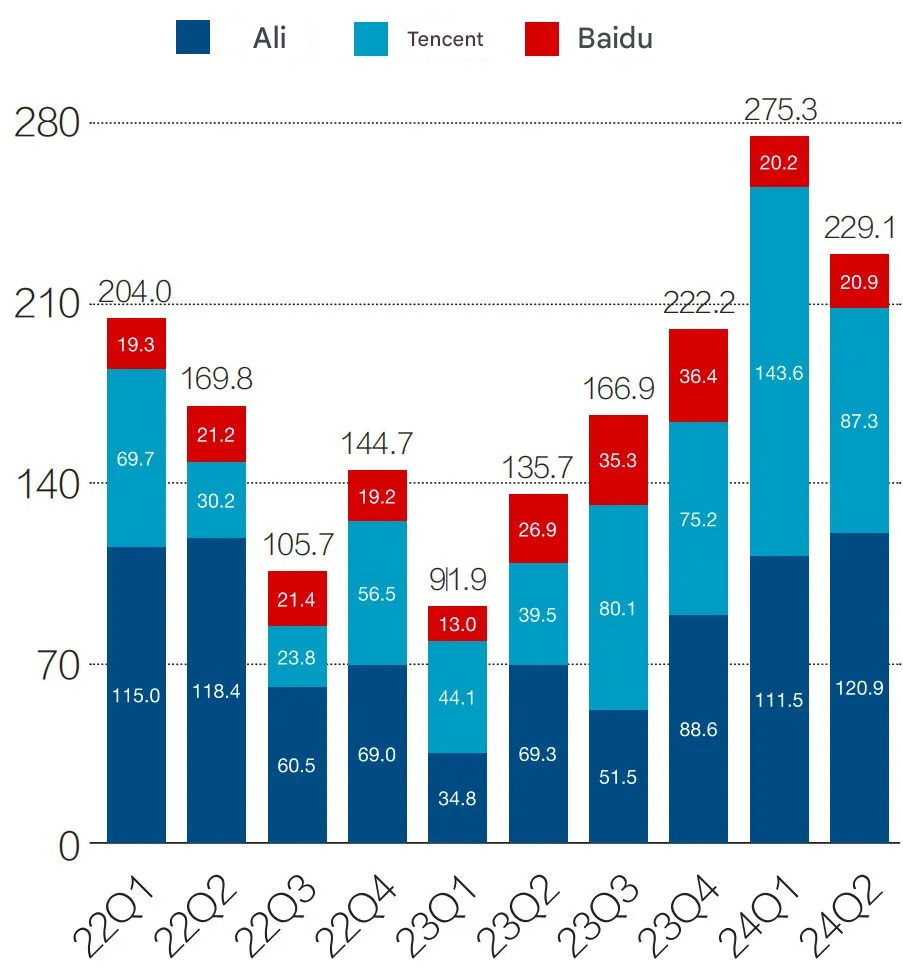
In the first half of 2024, Nvidia reported US$6.16 billion in revenue from the Chinese market (approximately 43.7 billion yuan), a year-on-year increase of 42.3%. Since domestic tech companies still largely rely on Nvidia chips, these figures provide another useful proxy for estimating the volume of chips purchased by major tech firms in China.
Figure 5: Nvidia’s global revenue scale in various regions (Q1 2022 - Q2 2024)
Unit: US dollars
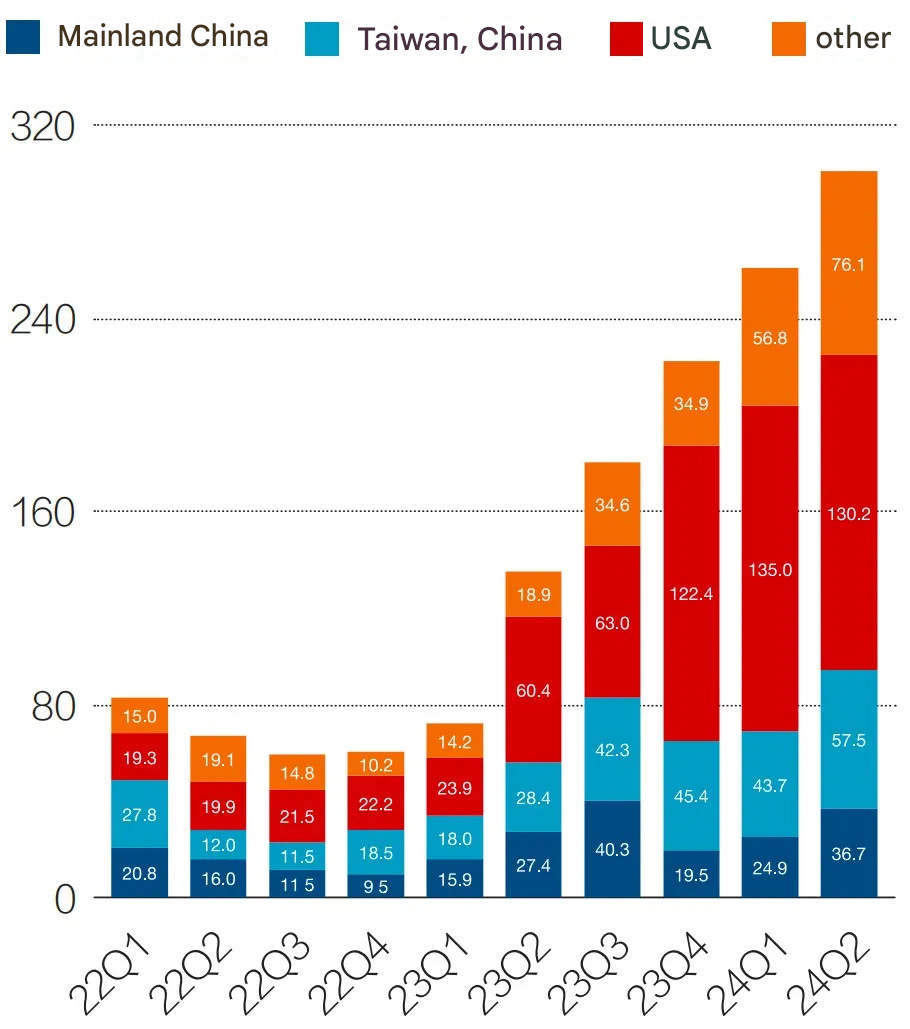
State-owned telecom operators
Caijing explains that since 2023, state-owned telecom operators have shifted focus from investment in 5G to computing center investments. In fact, the scale of their investment does not trail too far behind that of the private tech companies listed above.
China Mobile and China Telecom both disclosed 2024 computing-power investment plans, with China Mobile investing 47.5 billion yuan and China Telecom investing 37 billion yuan. The total of the two — 84.5 billion yuan — represents a year-on-year increase of 13%. China Unicom did not disclose this data, making data in this section incomplete as well.
Figure 3: Telecom operators’ computing power-related capital expenditures (2022-2024)
Unit: 100 million yuan
China Mobile has publicly released two procurement calls for public bidding. The two procurement documents show calls for more than 9,200 Huawei Ascend 昇腾 AI servers (an Ascend AI server is usually equipped with 4 to 8 GPUs, so this would include around 36,000 and 74,000 GPUs). The winning bidders are well-known domestic Ascend dealers, such as Kunlun 昆仑, Huakun Zhenyu 华鲲振宇, Powerleader 宝德, Baixin 百信, Changjiang 长江, Kuntai 鲲泰, Xiangjiang Kunpeng 湘江鲲鹏, and Sichuan Hongxin Software 四川虹信软件. Some articles suggest that China Mobile’s computing infrastructure built in 2024 relies 85% on domestic chips.
Caijing cautions that even these domestic chips rely on global supply chains:
A Huawei representative mentioned to us this September that although Nvidia’s H20 chips are still being sold to China for now, it is necessary to prepare for the worst-case scenario, as the supply chain could be disrupted at any moment. Huawei’s Ascend 910 series chips rely on high-bandwidth memory (HBM) supplied by South Korea's SK hynix. At present, domestic alternatives to HBM that are both effective and reliable are lacking. In an extreme scenario, if SK hynix’s HBM supply chain is cut off, the production capacity of the Ascend 910 chips would also be impacted.
For more on China’s international reliance on HBM, check out this recent ChinaTalk piece.
Local governments and SOEs
The data here seems to be most messy and incomplete. Caijing’s statistics show that as of October 16, 2024, there have been at least 30 bidding projects for AI computing centers launched in various cities, with a total investment of at least 27.5 billion yuan.
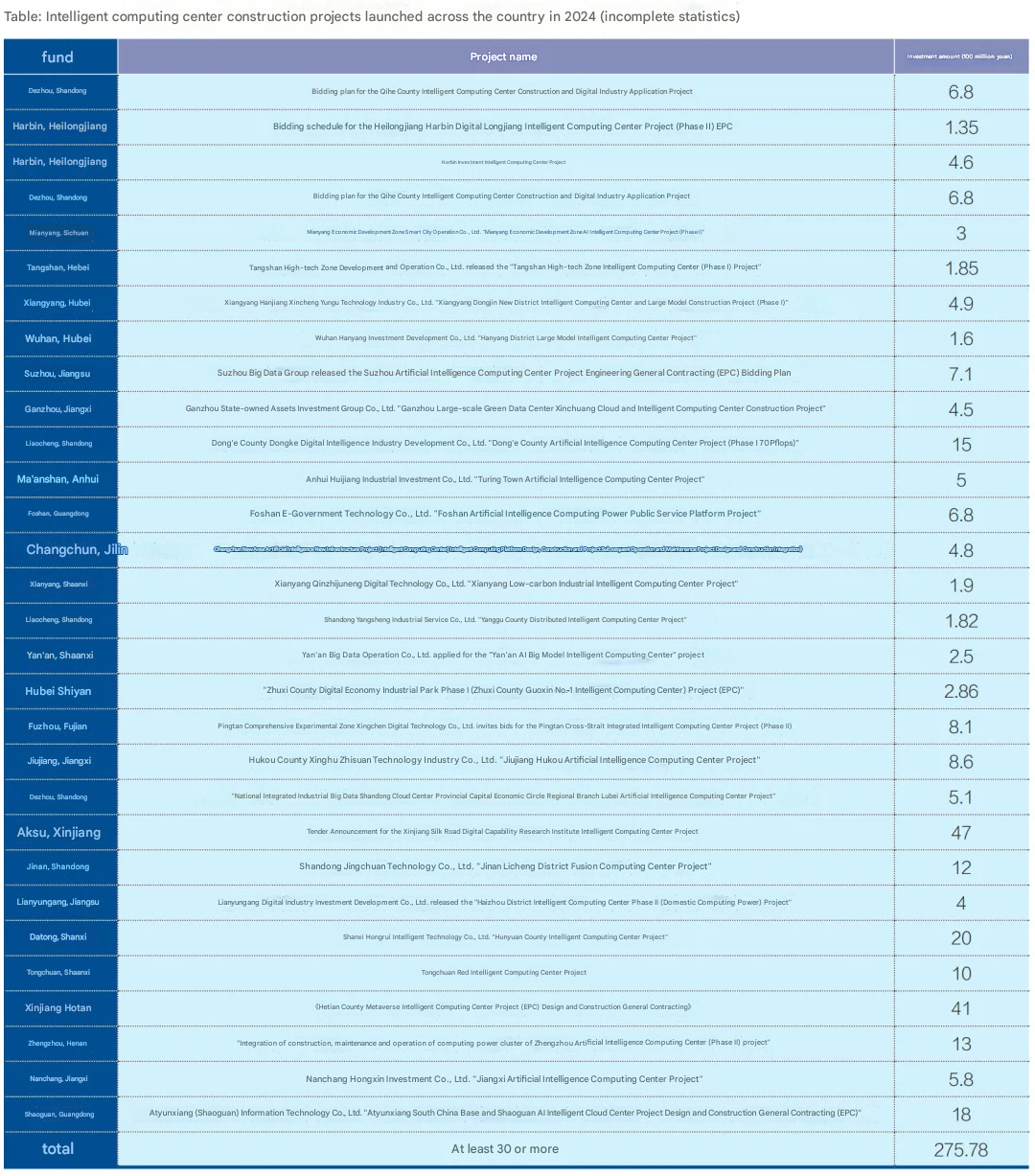
So how much compute do they have?
Based on the investment data provided above, Caijing provides a cautious estimate that China in 2024 has added
700,000 Nvidia H20 chips;
300,000 domestic Huawei chips.
The article also discusses a more optimistic estimate by Semianalysis:
more than 1 million Nvidia H20;
550,000 domestic (e.g. Huawei) chips.
According to Caijing, however, most Chinese industry insiders do not find the latter estimate credible.
In either case, this would leave China with enough GPUs to build several 100,000-GPUs scale clusters. For context, however, Epoch AI estimates that Google alone operates over 1 million chips. (It’s important to note that while Epoch AI’s figure below reflects total capacity, the numbers for China above refer specifically to the additional capacity acquired in 2024, making the two figures not directly comparable.)
TMTPost estimates that in H1 2024:
Domestic intelligent computing centers delivered 1.7 billion card-hours, with 560 million card-hours in use, resulting in a utilization rate of 32%. Other data shows that the average rack utilization rate in the computing infrastructure industry is currently less than 60%.
Utilization rates vary by operator:
Increasing the proportion of compute resources provided through cloud services can effectively enhance the efficiency of intelligent computing power. Alibaba Cloud and Huawei Cloud have already submitted this suggestion to multiple government departments.
A reasonable utilization rate of public cloud services is between 40%-60%, the utilization rate of government cloud services ranges from 25%-40%, but the utilization rate of privatized computing resources is generally no more than 5%.
Fake 10,000-GPU clusters?
Simply owning GPUs is not enough. There were many issues with efficient and rational allocation when China built its 10,000-GPU-scale clusters in 2023 and 2024.
An Lin 安琳, director of the Alibaba Cloud Intelligent Technology Research Center 阿里云智能科技研究中心, notes that there are currently three types of “10,000-GPU clusters” in China:
Fake 10,000-GPU clusters (假万卡集群): This refers to companies that technically own 10,000 AI accelerators, but the GPUs are distributed across multiple data centers in different locations. Each data center may only house a few hundred or a few thousand GPUs, and while the total number may exceed 10,000, they cannot function as a unified cluster.
Pseudo 10,000-GPU clusters (伪万卡集群): This type of cluster involves 10,000 AI accelerator cards deployed in a single data center. However, while the infrastructure exists in one location, these GPUs are not used as a unified resource for training a single large model. Instead, a portion of the GPUs might be used to train Model A, and another portion could be used for Model B.
True 10,000-GPU clusters (真万卡集群): A single, unified cluster housing more than 10,000 GPUs in one data center. These GPUs are interconnected and can operate as a cohesive unit through advanced large-scale resource scheduling technologies. This allows a single large model to be trained across all 10,000 GPUs simultaneously.
Creating a true 10,000-GPU cluster involves overcoming significant technical challenges, such as:
High-performance networking to handle enormous data flows;
Efficient scheduling systems to maximize GPU utilization;
Stable operations that can handle frequent hardware failures.
During the AI boom, many companies and local governments rushed to buy GPUs, assuming their mere ownership would ensure competitiveness in the AI race. Many of them lacked technical expertise to actually deploy them effectively, giving rise to “fake 10,000-GPU Clusters” and “pseudo 10,000-GPU clusters.” According to TMTPost:
Currently, stockpiling NVIDIA cards has indeed resulted in some computing-power waste. Many buyers lack the necessary networking, scheduling, and operational capabilities required for smart computing centers. A technical expert in the field bluntly stated, “There was too much speculative profiteering. Many people were not actually in this industry — they thought hoarding hardware could make money. They stuffed them into a random data center, but without solving issues like stability, fault tolerance, and other technical challenges. This caused a lot of waste.”
Some small cloud providers in China may provide cheap services simply because the quality is also low.
Government response
The Chinese government is acutely aware of the waste caused by chaotic data center construction in 2023 and early 2024. It has responded by trying to actively slow down the construction of new data centers.
According to TMTPost, the National Development and Reform Commission (NDRC) has stopped approving energy quotas for new data center construction. Exceptions may be granted if you use Huawei chips in one of the eight nodes of China’s “Eastern Data–Western Compute” 东数西算 project we explored in an article late last year.
A front-page article in the party-media outlet Science and Technology Daily 科技日报 — titled “The Construction of Intelligent Computing Centers Should Not Blindly Follow Trends” — made this argument:
The operation and management of smart computing centers rely heavily on professional technical talent and efficient management teams. Without these, the centers may fail to function as intended, potentially leading to idle equipment and wasted resources.
Therefore, deciding whether to build, when to build, and where to build smart computing centers requires scientific and prudent decision-making. A “herd mentality” or a rush to “follow trends” must be avoided. The overarching principle should be to build smart computing centers based on clear and sustainable market demand, appropriately tailored to local conditions, and with moderately advanced planning.
Local governments are now raising the bar for contractors:
Some local governments have strengthened requirements for the operation of intelligent computing centers. For example, a project in Dezhou 德州, Shandong, which is valued at approximately 200 million yuan, explicitly stated in the bidding documents that it would adopt a “design, construction, procurement, and operation integrated model” 设计施工采购运营一体化的模式. It requires an operating period of no less than five years and specifies minimum annual computing power revenue after the project’s acceptance and commissioning.
According to ZStack 云轴科技 CTO Wang Wei 王为, the government now has higher requirements for smart computing centers. Previously, it was enough to simply build the centers, but now they look for competent operators or integrate construction and operation to ensure the utilization of computing power.
Other local governments are trying to hand over their idle computing resources to cloud providers:
Some local governments have begun mediating to encourage cloud service providers to rent idle compute power from their smart computing centers. “We didn’t even know there were so many GPUs in the country. In a sense, the scarcity of compute power is accompanied by a mismatch of resources,” an industry insider said.
Several other government policies try to address resource waste in small and scattered computing centers:
The “Special Action Plan for Green and Low-Carbon Development of Data Centers” 数据中心绿色低碳发展专项行动计划 imposes strict and comprehensive requirements on the regional layout, energy and water efficiency, and use of green electricity in the data-center industry. The plan also proposed the “complete elimination of local preferential electricity pricing policies for high energy consumption.” People generally believe that this policy will accelerate the elimination of outdated capacity, improve the industry’s supply structure, and promote healthy development.
The Ministry of Industry and Information Technology (MIIT) recently issued specific pilot approvals for cloud-based smart computing services in six cities. These pilots aim to address issues related to the earlier construction of smart computing centers in various regions, particularly the waste of resources in small and scattered computing centers built with state-owned funds.
Apart from these government measures, the articles also suggest that as the market matures and investment strategies adapt, low-quality or inefficient actors (e.g., those deploying suboptimal or idle systems) may naturally be phased out. Over time, more efficient players will dominate, addressing some of the issues with idle computing resources.
Are Huawei chips especially likely to lay idle?
The articles provide mixed messages on the role of domestic chips in creating the paradoxes of idle computing power.
According to Caijing, the clusters most acutely affected by the paradox of idleness are the ones operated by state-owned mobile operators and by local governments. Part of the reason is that they are using domestic chips:
The situation with domestic AI chips is even more special. Currently, domestic AI chips have only achieved “usability” 能用, but are still some distance away from being “good to use” 好用. Blindly using them would only result in wasted computing power. Companies need to use various technical methods to adapt and maximize the efficiency of domestic AI chips.
TMTPost also describes the ecosystem challenges faced by Huawei chips, but quotes industry insiders as believing those are minor issues that are inevitable and will ultimately help the Huawei ecosystem to mature:
One side argues that domestic intelligent computing centers are still reliant on overseas ecosystems, requiring a transition period of three to five years. During this time, large-scale rapid construction of such centers is likely to lead to significant waste. … The expert remarked on the inefficiencies in domestic AI computing, observing, “Huawei’s operational capabilities are incredibly strong. However, before users were ready to adopt domestic GPUs or Huawei solutions, Huawei invested significant resources to develop computing clusters and intelligent computing centers. Telecom operators built clusters with tens of thousands of GPUs, but there’s still a gap between making the hardware available and actually using it effectively. As more domestic chips enter the market, these inefficiencies may become even more pronounced.”
The other side believes that overseas restrictions will only become more severe, and that the domestic AI computing ecosystem must mature faster. Compared to strategic national competition, some minor issues caused by overly fast construction are acceptable. … “That said, I’m optimistic about domestic GPUs overall, given the shifts in computing power trends in the era of large models. Previously, AI models were very fragmented, and Nvidia’s CUDA ecosystem was dominant because it had to support so many different models. Now, with large models becoming more consolidated, mainstream frameworks are more uniform. At the same time, Nvidia GPUs are extremely expensive, and with challenges in accessing their computing power, more people will be willing to experiment with domestic GPUs,” the expert added.
Transition from training to inference demand
In 2023, the rush of countless companies to develop foundation models spurred the construction of numerous computing centers across the country.
In 2023, there was a compute shortage because the “battle of the hundred models” led to a surge in demand for training compute. US export controls further restricted supply, and panic purchasing by companies exacerbated the imbalance between supply and demand. A strategic planner at a leading tech company mentioned in August 2023 that their company, in 2023, had purchased A100/A800 and H100/H800 chips on the market at prices 1.5 to 2 times higher than Nvidia’s official pricing, even acquiring scattered stockpiled chips from small and medium-sized distributors.
By 2024, however, many of these companies have abandoned their foundation model ambitions, leading to a sharp drop in training demand. This suggests increasing resource concentration on a few dominant players in the industry. According to TMTPost, out of the 188 models officially approved in China by October 2024 [ChinaTalk editor’s note: this data is unsourced in the original article, so take it with a grain of salt]:
Over 30% showed no further progress after approval;
Only about 10% were still actively training models;
Nearly 50% shifted their focus to AI application development.
Caijing agrees, noting:
The demand structure is changing. By 2024, compute became gradually sufficient as companies stockpiled more and more chips. The demand for model training began to slow, while the demand for application inference has not yet exploded. At this point, a transitional “gap period” emerged.
Currently, flagship models from domestic tech companies such as Alibaba, ByteDance, and Baidu have performance levels approaching OpenAI’s GPT-4. Since the next generation of models after GPT-4 has not yet truly emerged, the task of catching up with GPT-4 has largely concluded. As a result, major domestic tech companies have temporarily slowed their model training efforts.
Between 2022 and 2027, the proportion of training compute will decline to 27.4%, while the proportion of inference compute will rise to 72.6%.
However, inference demand is increasing. Baidu’s token consumption quadrupled from 250 billion in May 2024 to 1 trillion in August. ByteDance even reported a ten-fold increase for its model over the same time period.
Figure 7: Average daily token consumption of large models under Baidu and ByteDance
Unit: 100 million/daily average
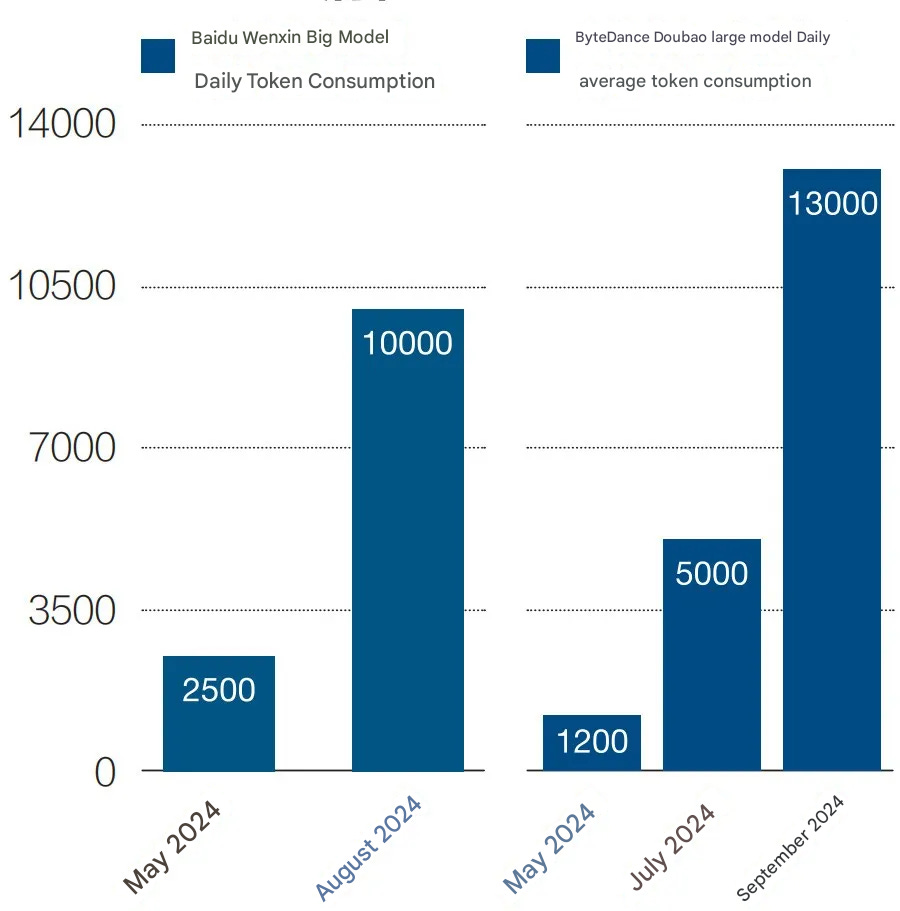
All of this suggests that there will eventually be shortages again. Caijing:
We have learned from multiple technology cloud service providers and some telecom operators that their common judgment is “sufficient in the short term, but insufficient in the long term.” In the short term, the existing computing power can meet basic business needs, with localized instances of overcapacity. However, in the long term, the implementation of AI applications will require more computing power.
The length of this “transition period” depends on two factors: first, when the race to train the next generation of models begins; second, how quickly inference compute demand grows, which depends on the speed of AI application adoption and penetration.
Some technical experts in tech companies predict that this transition may last until mid-2025, but it will not be very long overall.
Hi, I'm having a hard time interpreting the 2nd figure in the article (Nvidia's revenue in various regions). You say: "In the first half of 2024, Nvidia reported US$6.16 billion in revenue from the Chinese market (approximately 43.7 billion yuan), a year-on-year increase of 42.3%". However, for Mainland China:
Q1 2024: 24.9
Q2 2024: 36.7
which gives a total of 61.6 for the first half of 2024. So is "US$6.16 billion" a typo in the decimal place?
You also say: "For statistical convenience, Nvidia’s revenue from mainland China, Taiwan, and outside the United States is combined as “others” in the chart.". Looking at e.g. Q2 of 2024, we have:
Mainland China: 36.7
Taiwan: 57.5
so the 'other' category should be at least 94.2, but in the chart it is 76.1.
Could you please clarify these two points? Thank you.