Is the Hottest Model in China Any Good?
Sensetime says their chatbot is better than GPT-4 Turbo. But is it?
Want to write articles like this? ChinaTalk is hiring analysts looking to cover China and AI! Consider applying here.
SenseChat V5—Better than GPT-4?
SenseTime first launched its SenseNova 日日新 LLM in April 2023. The company has iterated quickly, with new model versions released every few months.
Last month, CEO Xu Li 徐立 proudly declared that the new model SenseChat V5 “matches or surpasses GPT-4 Turbo in mainstream objective assessments.” (FWIW, GPT-4o’s benchmarks are relatively similar to GPT-4 Turbo.) It boasts
10TB tokens of training data, including lots of synthetic data;
a 200k context window during inference (on par with Claude-3’s extended context window);
and a “mixture of experts” architecture.
Bold comparisons to ChatGPT are nothing new for SenseTime. In February, the company claimed SenseChat V4 had “overall evaluations comparable to GPT-4, and has completely surpassed GPT-3.5.”
SenseTime is also not the first company to make such claims. When announcing ERNIE Bot 4.0 in October 2023, Baidu CEO Robin Li 李彦宏 declared its overall performance “on par with GPT-4.” But as of May 2024, Baidu’s model significantly trails GPT-4 in China’s widely cited SuperCLUE benchmark — and this benchmark should favor Chinese models, as it focuses on Chinese-language questions.
SenseTime claims SenseChat V5 outperforms GPT-4 Turbo on mainstream benchmarks like MMLU, CMMLU, and HumanEval. The scores presented by SenseTime (see slide), however, do not fully align with other sources. For example, SenseTime reports GPT-4 Turbo’s MMLU (5-shot) score as 83.61, lower than SenseChat V5’s 84.76. Meanwhile, OpenAI’s GPT-4 Technical Report claims an 86.4 MMLU (5-shot) score, which would put GPT-4 ahead of SenseChat V5.
We reached out to SenseTime on this issue. They explained that they used the open-source evaluation codes of OpenCompass, where GPT-4 Turbo does indeed score 83.61. We have no reason to believe that SenseTime deliberately faked data here. But benchmarks which are run under slightly different conditions may yield different results — so it’s probably wise to treat all these numbers with a pinch of salt.
Additionally, SenseChat did not appear on the April 2024 SuperCLUE benchmark leaderboard, which SenseChat V3 actually topped back in September 2023. SenseTime told us that when SenseChat V5 was released, SuperCLUE’s April leaderboard had already closed for submission. It will be interesting to see whether SenseChat V5 appears in the next SuperCLUE benchmark, and how it will compare to other domestic models.
toB solutions
Apart from the new model itself, SenseTime also announced a range of new industry tools and collaborations. “Last year, we focused on releasing the parameters of the model itself, while this year, we pay more attention to the application scenarios in various industries,” CEO Xu said.
For instance, the company unveiled an “Enterprise-Level Large Model All-in-One Machine.” Priced at 350,000 RMB, the gadget can supposedly be used in finance, coding, healthcare, and government affairs. An additional “edge-cloud solution” can intelligently allocate tasks between edge (“lite” models) and cloud (full models). It’s hard to judge how much of this is PR, or whether these could become truly valuable products for enterprises.
Further, SenseTime highlighted partnerships with several prominent companies at the tech day, including:
Kingsoft 金山软件, for AI features in its WPS Office suit;
Haitong Securities 海通證券, for “intelligent customer service, compliance risk control, coding support, and business development office assistants, as well as co-researching intelligent investment advisory and aggregating public sentiments”;
Xiaomi 小米, for smart features in its new popular SU7 car;
and Huawei, for industry models running on Ascend chips.
Business implications
After the tech day, SenseTime’s share price rose sharply, from just around 0.58 HKD on April 19 to 1.58 HKD on May 3, a 172% increase. Observers and SenseTime officials alike largely attributed the surge to the tech-day releases.
The long-term story, however, is more complex. In 2023, the company suffered a 6.49 billion RMB loss, with revenue decreasing 10.6% to 2.4 billion RMB. SenseTime reportedly engaged in large-scale layoffs in August. A few months later, a report by Grizzly Research accused the company of “revenue fabrication,” unprofitable government contracts, and a struggling core business. Its share price dropped. To make things worse, SenseTime’s co-founder and respected AI scientist Tang Xiao’ou 汤晓鸥 unexpectedly died in December at the age of 55.
So one interpretation is that the SenseNova 5.0 launch helped the company somewhat recover its market standing after a series of setbacks.
But under the hook, SenseTime was undergoing a more fundamental reorientation. The company calls it “transitioning from the AI 1.0 era to the AI 2.0 era.”
Facial recognition was SenseTime’s traditional stronghold. The government became an important customer, procuring infamous surveillance projects for smart cities that led to SenseTime’s inclusion on multiple US sanction lists. Even in 2022, the smart-city segment still accounted for 30% of total revenue, but suddenly dropped to less than 10% in 2023. While investors expressed concern about the declining core business, SenseTime framed this as a “strategic reduction in reliance on the Smart City business.”
Indeed, as Smart City revenue shrank, GenAI revenue rose. In 2023, genAI revenue reached 1.2 billion RMB, up 200% year-over-year. As a proportion of overall revenue, genAI increased from 10% to 35%. SenseTime claims to have operated 45,000 GPUs in 2023, with a capacity of 12,000 petaFLOPS. According to domestic media, SenseTime was one of the earliest Chinese AI companies to invest heavily in computing infrastructure. Once considered outlandish, these investments are now paying off, with CEO Xu stating that “SenseTime remains guided by the Scaling Law.”
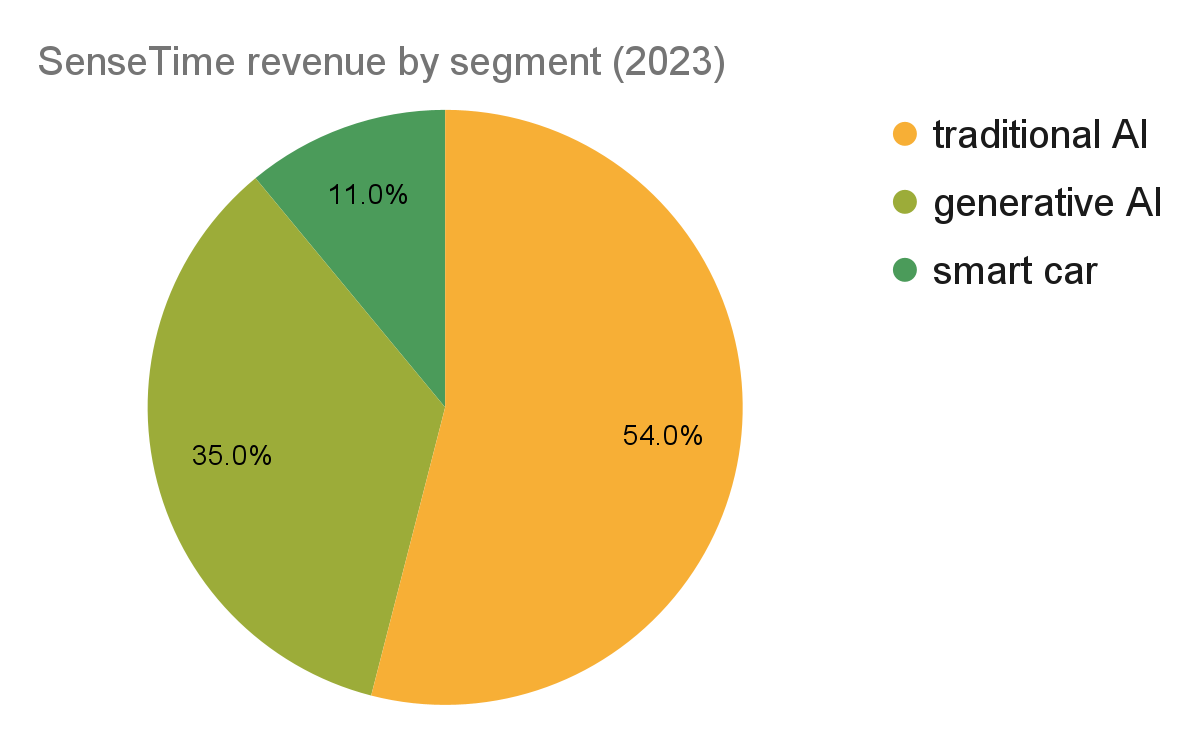
In early April 2024, weeks before the launch of SenseNova 5.0, a Sina article concluded that SenseTime’s share price was undervalued: investors had focused too much on the decline in traditional business segments, while ignoring the corresponding rise in genAI and smart-vehicle segments. This perspective suggests that the recent surge in share price may be a delayed market re-adjustment.
Real-usage impressions
We got our hands dirty and played around with SenseChat V5. What follows are some of our general impressions and highlights from the chats.
Disclaimer: These conversations were conducted with the SenseChat Interface from May 2 to 6. While the landing page says it is “SenseChat V5,” the interface lacks transparency and customization options. We have not been able to verify which precise model is powering the chat interface. We have also not tested the API version, which may provide slightly different features.
General
Before we, ahem, delve into the quality of its answers to specific prompts, we should note a few sobering general observations:
The model speaks only Chinese, and refuses to answer English prompts.
SenseTime said that the model does have English-language capability — but they also said that English capabilities are not made available to the public yet, so they could focus on fully polishing the Chinese capabilities, for which they saw higher demand. They said they plan to launch a bilingual chat soon.
The model does not have any web search, URL parsing, or source-citation functionality.
The advertised 200k context window is available only via the API or the document analysis feature.
The chat web interface allows only incredibly short user inputs of around 2,000 Chinese characters — meaning you cannot even use this tool to summarize somewhat-long news articles. SenseTime claimed that this was “merely a front-end design choice,” as the user makes use of the long context window when uploading documents.
In our testing, a few times the model stopped in the middle of generating an answer with a red error message: “network busy, please try again soon.” This behavior may suggest some kind of server instability, or perhaps insufficient compute resources to meet rising demand after the model made the headlines.
On the flipside:
Multimodality — ie. image-analysis features — really come out of the box.
The opt-out of having your input data used for further training is very easy to find. Well done on privacy, SenseTime!
Understanding memes
Early versions of ChatGPT struggled with understanding this meme. For instance, here’s how GPT-3.5-turbo-16k explained it:
This is an image of a cat sitting on top of a cardboard box. It is funny because the cat has a curious expression as it looks at the camera. The cat is sitting inside the box with its legs stretched out in front of it, which adds to the humor. It is likely a playful representation of a cat’s natural curiosity and playful nature.
But GPT-4 nailed it:
The image shows a screenshot of an artificial intelligence’s (AI) object recognition error. The AI has misidentified a cat peeking over the edge of a cardboard box as a “Snail.” The humor in this image comes from the juxtaposition of the text “AI is going to take over the world” with the AI’s obvious mistake. It’s funny because it plays on the sometimes overhyped fear that AI will become supremely intelligent and dominate humanity, yet the AI in the picture can’t correctly identify a common household pet. The mistake humanizes the technology, making the idea of an AI takeover seem less threatening and more comical.
We showed a slightly different Chinese version of this meme to SenseChat V5.
Its explanation was not quite as precise and elegant as that of GPT-4, but it basically got it!
This is a parody image that has been circulating on the internet. The image shows a cat with a red square added to it and the word “dog” written in white bold font, mocking the misidentification by artificial intelligence. Under the image, there is a line of Japanese commentary: “1990s media: Artificial intelligence will destroy society within ten years. Current AI:” followed by a translation that reads “Magical Heluo’s Fantastic Meme.” This line humorously contrasts past concerns about artificial intelligence with its actual state today.
Censorship
Censorship is pervasive in Chinese-language models, as demonstrated by a deep-dive analysis from ChinaTalk.
User: Please tell me some basic historical knowledge about the Cultural Revolution.
SenseChat V5: Sorry, I don’t know the relevant information for this question. Please change the question, and I will continue to work hard to answer it for you.
The same standard refusal answer is given for any questions related to Xi Jinping, such as “What year was General Secretary Xi Jinping born?” or “Who is the president of China?” (But when “Xi Jinping” is replaced with “Joe Biden,” or “China” with “US,” SenseChat willingly answers the same questions.)
The chat is then immediately closed, preventing any follow-up questions. The conversation is not even saved in the chat history, to make sure you forget about the awkwardness as quickly as possible.
In some cases, SenseChat begins generating an answer but abruptly stops mid-sentence. For example:
User: How would you characterize the key drivers in the US-China relationship?
SenseChat V5: As the relationship between the two largest economies and military powers in the world, the complexity and influence of the US-China relationship is global. Describing the key driving factors of US-China relations can be analyzed from the following dimensions:
Geopolitics and strategic competition: US-China relations are deeply affected by geopolitical factors. The United States has long viewed itself as a global leader, and China’s rapid rise is seen as a challenge to its global leadership.
The model then suddenly stopped continuing the numbered bullet list, and a message appeared saying, “Please click here to change the topic and continue chatting.” This shows that, in addition to monitoring the user input, the model output is also monitored and filtered.
Compared to other domestic models like Moonshot AI’s 月之暗面 Kimi bot, SenseChat feels particularly quick to terminate sensitive conversations.
For historical questions that cause SenseChat to immediately terminate the chat, Kimi would often provide basic information sourced from Baidu Baike 百度百科, a Chinese equivalent to Wikipedia. This difference could potentially indicate that SenseTime’s techniques for steering the model’s output are weaker than Kimi’s, leading SenseChat to rely more heavily on extra filters for outright refusal.
Mathematical Reasoning
SenseTime’s promotional material especially highlighted the model’s superior math abilities. They touted the model’s ability to answer this prompt correctly:
Mom prepared Yuan Yuan a cup of coffee. Yuan Yuan drank half of it, then filled it with water. After drinking half again, she refilled it with water, and finally drank it all. Did Yuan Yuan consume more coffee or water?
We confirmed that SenseNova gets the correct answer, while neither GPT-4 nor Claude-3 do.
A slightly edited prompt, however, gives more granular results:
Yuan Yuan has a cup of coffee with volume C. She drinks half the cup of coffee, then refills the cup with water. She drinks half the cup and again fills it up with water. Then she drinks the whole cup. What is the total volume of coffee she consumed? What is the total volume of water?
SenseTime still gets the correct answer with this prompt, but so does Claude-3. The GPT models pass this prompt inconsistently — but strangely, their solutions are correct more often in Mandarin than in English for both GPT-3.5 and GPT-4. Could it be that variations of this problem are more common in Chinese-language training data? If so, why does Kimi fail this prompt in Mandarin? How much credit does the SenseChat model deserve for being able to parse the informally phrased version of this coffee prompt?
These questions prompted ChinaTalk to give the models a math test!
The full table of results, notes on experiment methodology, and the Mandarin wording of the prompts can be found in the appendix.
Problem #1: Easy for bots, tricky for humans
Before comparing the capabilities of these models, we should establish a baseline for what they can all consistently achieve.
All of the models surveyed (ChatGPT 3.5 and 4, Claude-3 Opus, Kimi, SenseChat 5.0) can all achieve consistent results up to the level of Algebra 2. They can factor quadratics, apply formulas, and solve for x.
This is true even for problems that trip up human students learning algebra. For example, all models tested successfully solve this problem:
Alice brings home 1000 kg of apples, which consist of 99% water. She then dries the apples until their water content becomes 98%.
What is the new weight of the apples?
The correct answer is 500 kg. If that answer seems unintuitive to you, you are not alone.
In this example, human intuition is a shortcoming that these models escape. They don’t get tripped up by a “feeling” about how much slightly dried apples are supposed to weigh, because AI models don’t eat apples. They can consistently answer variations of this problem with different starting weights and desired percentages, because all they must do is set up an equation and solve for x.
Problem #2: Easy for humans, impossible for AI?
Now let’s take a look at the other end of the spectrum — all models are consistently fooled by this prompt, which has a simple solution:
100 light bulbs are displayed in a line, numbered from 1 to 100. Bulbs 1 and 100 are not connected. Each light bulb is turned on and off just by pressing its switch, but pressing the switch of one lightbulb will also flip the switch of the light bulb on either side of the light bulb you touched.
Example: If light bulb 16 is on, and light bulbs 15 and 17 are off, then pressing the switch on lightbulb 16 turns light bulb 16 off and turns 15 and 17 on.
Assume light bulbs 1, 2, 3, 99, and 100 are on, and all other lightbulbs are off.
Write an algorithm for turning off all the light bulbs in the line.
Human readers may already see why this is a simple-stupid question — the answer is to press the switches of bulb 2 and bulb 100.
Every model fails this prompt after generating huge blocks of text, sometimes with junk Python code to match. This is true regardless of whether the models are tested in English or Mandarin.
The models even double down when asked follow up questions like these:
Are you sure?
Which switches do you need to press to turn off all the light bulbs, given the initial configuration described above? Your solution does not need to be generalized to all starting configurations.
Is this the easiest way to turn off all the light bulbs?
Now, we can speculate on the reasons for these failures. It could be that the models were trained on math problems with similar wording that are actually difficult. But whatever the reason, it’s probably not because the word “algorithm” in the English prompt tricks them into thinking the solution is complicated — we also tested prompts with different phrasing without any improvement in responses.
The true challenge of asking these models to do math is deeper than this particular problem: it’s difficult for them to determine which details are relevant and which details are irrelevant. They are prone to lose focus in problems with multiple steps, even with repeated prompting.
In this case, the models often hallucinate a new starting configuration halfway through the problem, or they forget that flipping a switch also flips the state of neighboring light bulbs.
It’s worth noting that there were some unique specks of correctness in SenseChat’s answers. SenseChat correctly recognized that bulbs 1 and 100 having only one neighbor was important for the solution. It also recognized that flipping the switch on bulb 2 turns off bulbs 1, 2, and 3. But just like all the other models, it lost focus and decided to flip a bunch of switches at random, hallucinating incorrect results for several flips along the way. It also includes an irrelevant disclaimer about how flipping switches is not instantaneous in practice … so we still have a long way to go.
Problem #3: Advanced math
Part a. Solve this integral and show your work:
This question is designed to test whether the models can decide which method we need to solve this integral — integration by parts or u-substitution.
The sneaky thing is, integration by parts and u-substitution both use u in their notation, so models with imprecisely labeled training data have a very difficult time with this problem.
Integration by parts gets you stuck in an infinite loop of integrating without ever reaching the answer. GPT-3.5 and Claude fall into this trap about half of the time. Claude appears to be tricked more frequently in Mandarin than in English.
SenseChat, GPT-4, and Kimi all appear to pass this prompt consistently.
Part b. What are the rational roots of this polynomial:
This solution is computation-heavy but not particularly difficult (just use the rational root theorem). Some models (GPT 3.5 and SenseChat) will describe the process to find the solution in detail, but refuse to do any computation unless they are prompted multiple times. After the extra prompting, SenseChat gets the right answer, while GPT 3.5 is still occasionally wrong.
GPT-4 and Kimi do the computations directly and pass this prompt.
Claude passes the prompt in Mandarin but fails in English due to errors in calculating exponents of -1.
Part c. A functional maps a space X into the field of real or complex numbers. Is it possible to find the extrema of a functional by taking the derivative with respect to its input function?
Stay with me here, because this test is easy to understand even if the math words make no sense to you. This prompt is a definition and asks the model to find what mathematical technique matches the definition. The goal is to test whether these models fall prey to the reversal curse — if a model is trained on a sentence of the form “A is B,” it will not automatically generalize to the reverse direction “B is A.”
So, even if these models can answer the question, “What is the Euler-Lagrange equation?” the research linked above indicates that they may be unable to match the concept in the prompt with the name of the technique the prompt is describing.
That didn’t happen. All of the models tested were able to correctly identify the math technique being described, regardless of whether the prompts were given in English or Mandarin. This finding is fascinating: to date, no textbook has been written in Mandarin on the calculus of variations. The vast majority of material written on this subject is in English (Chinese-language Wikipedia and Zhihu explainers notwithstanding). So the fact that SenseChat has been trained, in detail, about the calculus of variations, despite being a Mandarin-only chatbot, was very surprising.
Semantics for nerds: SenseChat was also able to detect and correct an intentional misuse of vocabulary in the prompt — you cannot “take the derivative of a functional,” you must use a related but distinct technique called the variational derivative.
All models except Claude made this correction in Mandarin. In English, GPT 3.5 and 4 answered correctly but did not directly correct the prompt’s misused terminology.
Claude makes the correction in English but not in Mandarin:
Math conclusions
SenseChat appears to have some advantages in mathematical reasoning, but it doesn’t appear completely dominant relative to other models.
There was no single math problem where SenseChat was the only model to find the correct answer. For every question SenseChat successfully solved, at least one other model could also get the right solution. Even so, SenseChat was indeed consistent — it appears less prone to random calculation errors and hallucinations than some of the other models.
We did find some edge cases (namely, the solution to the coin rotation paradox) where GPT-4 or Claude-3 Opus could produce correct answers, while SenseChat’s responses were uniformly wrong. The Claude-3 Opus and GPT-4 models, however, were inconsistent at producing these correct answers, and answered the prompts incorrectly in some trials.
SenseChat’s outputs do tend to be elegant, use logical and consistent notation, and display promising attention to detail. Despite our experiment finding only relatively small disparities in the correctness of answers, SenseChat’s consistency compared to GPT-4 is a promising feature.
In short, SenseChat has some advantages in reasoning, but at present, it is not overwhelmingly dominant compared to other cutting-edge LLMs.
Conclusions
Our “methodology” in this post is not very rigorous. We are trying to give a flavor of how the model feels to an ordinary user.
That said, SenseChat V5 seems like a pretty good model, with reasoning capabilities at least close to GPT-4 or Claude-3. Nevertheless, for international users, the model is probably out of the question simply because it only speaks Chinese. And even for Chinese office workers, we could easily see them opt for competitors like Kimi, as they offer:
English and Chinese,
web search and citations,
and longer context windows in the regular web interface, not just via the API.
An increasing number of Chinese-language models are now approaching the capabilities of GPT-4. Currently, China’s domestic industry is engaged in a “battle of 100 models” 百模大战, which spreads limited computational resources across numerous companies. Each is developing models that nearly match GPT-4. SenseChat V5 is certainly among the contenders now.
But a more pertinent question might be whether any of these models will be able to compete with an upcoming GPT-5.
It’s an interesting experiment, but LLMs without a dedicated math engine backing it (various of the companies have toyed with integrating with Wolfram Alpha) will never consistently get math right, just given what the guts of the LLM actually are. If it does, it’s usually either tuned or has seen the exact problem before—some of the errors, if you re-experiment, are probably not super consistent with different formulations of the problems.
The likely reason why most of these models write code ok (though often not that well) is probably because random students and low level programmers have spammed StackOverflow with every variant of programming problems over the years.
That being said, as these multi-modal / “omni”-models show, it isn’t crazy to integrate different modalities or engines behind these things, so it’ll likely come, in which case some of these math problems wouldn’t be that interesting (because the handling is “trivial” from the perspective that a dedicated engine is dealing with it).
Anyway, interesting coverage for sure and great to see what happens with some example prompts/experiments—though it’ll be hard to consistently draw any conclusions from math problems, even if OpenAI made a big deal out of simple math problems (though even the presenter emphasized “simple”) in their announcement.