TikTok, Congress Takes a Massive L on S&T Funding, AI Hardware Controls
Zhang Yiming and the future Beijing closed off, Congress being Congress, and control at the chip level not a panacea
What to Do about TikTok
The following is an excerpt from a piece I wrote in 2020, back when Trump was talking about banning TikTok.
Chinese tech firms are not enthusiastic partners in foreign-policy endeavors, and aside from the occasional offer of free office space and tax benefits would generally prefer to have nothing to do with the government. Take DiDi’s initial response to police requests for data — in one instance, after twice outright refusing the request on privacy grounds, it finally simply printed out a few boxes of documents that for the police’s purposes were nearly useless. ByteDance’s CEO is surely not happy to have to issue apology letters and face mandated shutdowns of popular products.
Honestly, I feel bad for Zhang Yiming 张一鸣 and the rest of the new generation of Chinese tech founders.
I’ve read at least a dozen Chinese-language profiles or Q&As with him. These articles, coupled with personal interactions with a handful of other prominent tech new-generation CEOs, have given me a sense of their personal ambitions. They were promised a China opening to the West, were all active online back when the Chinese internet was much more open than it is today, and many spent years in Silicon Valley working for top American tech firms. For them, to compete against Western firms overseas is a natural evolution after saturating a domestic market, as well as the ultimate challenge for leaders who have built their companies aping the org structures and speed of Facebook circa 2009 to face off against the real deal.
The proper mental model of a Chinese tech CEO under 40 is not that of a faithful Party member toiling ceaselessly to spread Xi Jinping Thought, but rather a nerdy engineer who worked in the Valley and daydreamed of building something as big as Sergey and Mark.
Like tech titans in SF, most would much rather have nothing to do with politics, and on the whole, are much more liberal than your average Politburo member. I have less confidence, however, in extending this model to Huawei and its CEO Ren Zhengfei 任正非, who comes from an earlier generation.
But at the end of the day, there is very little that these firms can do to push back in a Party-state environment. China’s national intelligence law 国家情报法, according to one interpretation, gives total authority to the government to compel firms — and with no independent judiciary, even extralegal pressure is very hard to resist. CCP regulators who can take massive bites out of market capitalization at will, to say nothing of threatening the personal security of senior tech leadership that doesn’t follow their suggestions, make keeping officials at home happy ByteDance’s first priority, regardless of the business risks abroad.
S&T Spending In the US vs. China—Congress Decided to Take a Massive L
The bigger story this week than TikTok — in terms of long-term national competition — may be Congress not stepping up to the plate and appropriating what it authorized for research, development, and technology policy implementation. Below, Divyansh Kaushik of the Federation of American Scientists walks us through his meme.
China’s recent Two Sessions 两会 — the annual convening of the National People’s Congress (NPC) and the Chinese People’s Political Consultative Conference (CPPCC) — highlighted an ambitious vision. Despite economic challenges, a striking 10% increase in spending on science and technology research underscores Beijing’s determination to break free from foreign dependencies, especially in areas vital for future dominance such as artificial intelligence (AI), semiconductor manufacturing, and quantum computing.
Here at home, meanwhile, Congress’s recent budget appropriations for Fiscal Year 2024 tells a story of missed opportunities. The budget slashes funding for the National Institute of Standards and Technology (NIST) by approximately 12% and the National Science Foundation (NSF) by over 8%. These cuts come at a precarious moment: NIST is already grappling with significant operational challenges, from decaying infrastructure to a pressing need for additional personnel. Instead of fortifying America’s position as a global leader in technologies of the future through strategic investments in R&D, Congress has decided to play a dangerous game of penny-pinching.
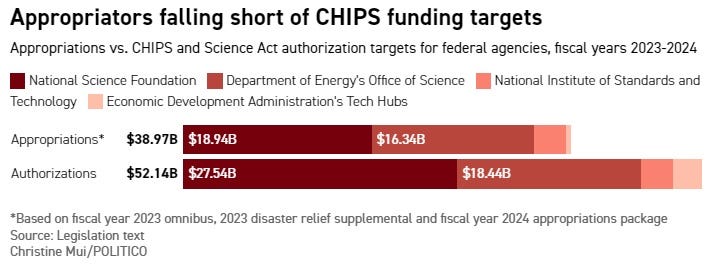
These reductions severely limit the United States’s capacity to respond to President Biden’s Executive Order on AI and for these agencies to fulfill other Congressional mandates required in the CHIPS and Science Act. At a juncture where emerging technologies — particularly AI — are critical battlegrounds for global leadership, such fiscal decisions are tantamount to self-sabotage. Unfortunately, that has been a common theme of the 118th Congress: strong rhetoric without the funding to back it up. To ensure America’s continued leadership and secure its interests against rising challengers, Congress must realign its appropriations with the strategic imperatives of the age and recognize that technology is at the core of national competitiveness.
What AI Hardware Controls Can and Can’t Do
Lastly, we’ve got a piece from Lennart Heim that helps rightsize expectations around AI hardware controls.
Introduction and Background
Rapid adoption of AI hardware controls without sufficient scrutiny could lead to outcomes that are counterproductive to the intended goals.
The benefits of implementing hardware mechanisms, as opposed to software or firmware alternatives, warrant clear articulation:
While certain controls could be achieved with software solutions, hardware-based approaches often offer potential superior security due to their increased resistance to tampering.1
These mechanisms are enforced upon the acquisition of the chip, establishing built-in controls from the start.
It is equally important to evaluate the assurances provided by hardware mechanisms, weigh their associated costs, and consider the security implications they introduce — extending beyond mere technical considerations. For example, highly invasive mechanisms, such as a US-controlled “remote off-switch,” could significantly deter the widespread use of these chips, potentially raising concerns even among NATO allies about dependence and control.
Below, I outline four considerations and limitations of hardware-enabled mechanisms:
Clarifying Assurances and Strategic Applications of Hardware-Enabled Mechanisms
Mechanisms are Vulnerable to Circumvention
Prolonged Lead Times in Hardware Mechanism Rollouts
Broader Considerations for Compute Governance
I. Clarifying Assurances and Strategic Applications of Hardware-Enabled Mechanisms
The discourse surrounding hardware mechanisms for AI chips is often oversimplified toward a binary understanding of AI systems, suggesting a straightforward mechanism capable of discerning “good” AI from “bad.” This is reductive, overlooking the complex nuances that differentiate desired AI applications from those deemed undesirable. The challenge extends beyond this categorization, where the implications of technical parameters on AI’s development and deployment become a central consideration.2
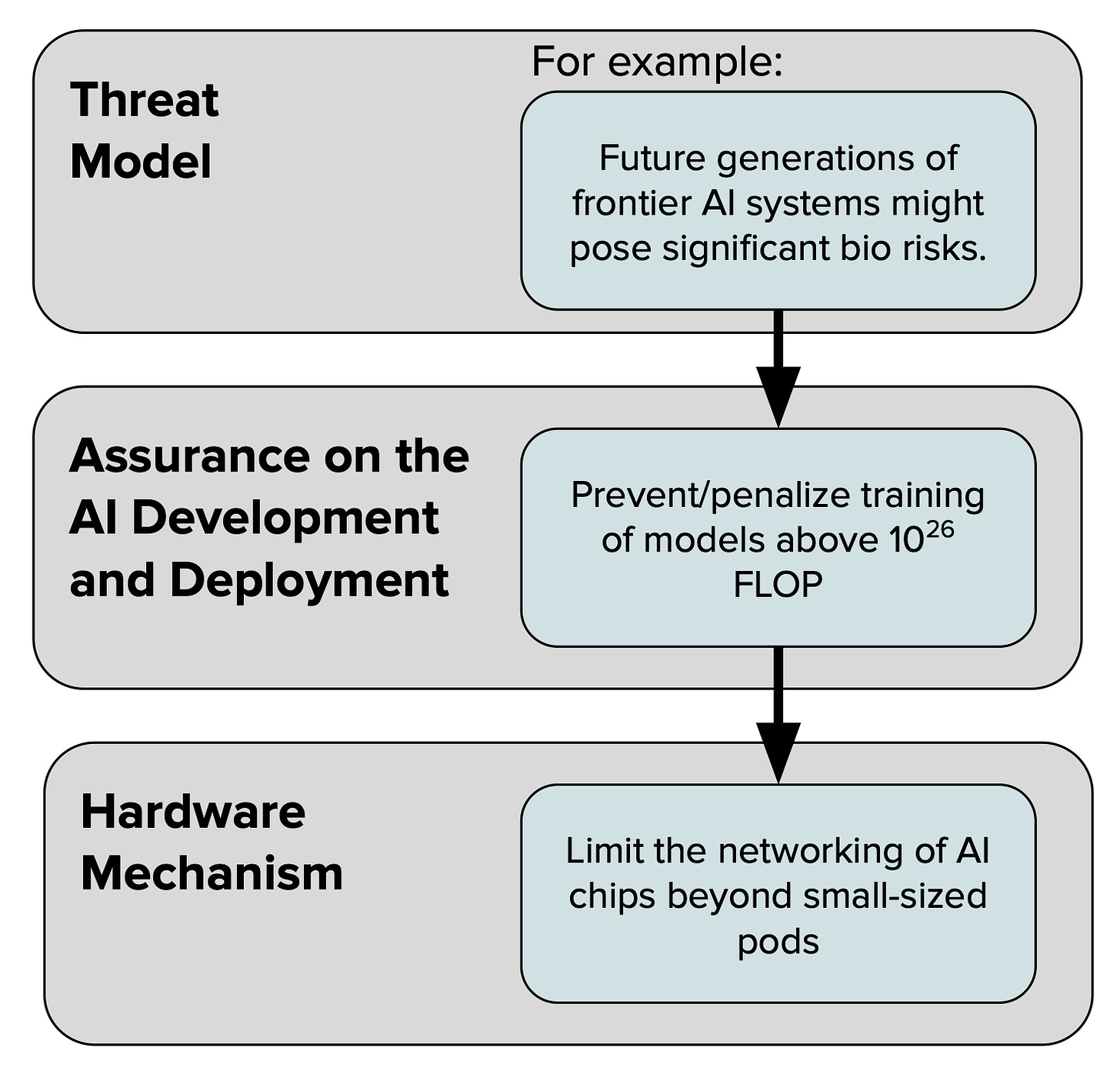
(a) First, identifying the types of assurances we seek from AI hardware mechanisms requires a comprehensive AI threat model. Such assurances might range from influencing the cost of AI model training to delaying deployment, increasing compute costs or even specific constraints like preventing chips from training models on biological data or beyond a certain computational size. Recognizing these diverse requirements is crucial, as each assurance might necessitate distinct mechanisms.
For instance, while halting an adversary’s ability to train a model comparable to GPT-4 may be a common desire, it is essential to understand that such interventions typically impose additional costs rather than an absolute stop (a “cost penalty” or “imposition”). Therefore, the question often becomes how much more onerous we can make it for them to develop such models. Moreover, the focus should extend beyond the one-time action of training. It should consider the deployment and diffusion of AI systems throughout the economy, which are influenced by compute capacities and, arguably, easier to impact than preventing the training of a system entirely. If an entity can train an advanced system — assuming it has already monetized other systems, found substantial economic adoption, or learned research insights from existing deployments (all of which are facilitated by compute) — then the control of the total compute available and its cost become even more important.
(b) We must examine how these desired assurances align with current strategies, such as export controls or outright bans on certain chips. What advantages do hardware mechanisms offer over these existing approaches? For instance, do they enable less intrusive governance that allows for continued marketability of AI chips? Are they a precursor to domestic regulations that would necessitate the deployment of certain hardware features? There is a real possibility that even with successful hardware mechanism deployment, a bifurcation of product lines may arise, with “on-demand” products catering exclusively to certain markets, potentially undermining the broader goal of universal hardware safeguards.
In considering the target chips of hardware mechanisms, we should deliberate on which devices to target. Should they be applied to chips within regulatory “gray zones,” to consumer GPUs currently exempt from controls? Or should they enable the export of chips previously above the threshold under new regulatory conditions?
(c) The broader AI governance strategy that surrounds these hardware mechanisms needs careful consideration. Which existing governance frameworks can support the effective functioning of these mechanisms, and which new strategies might be required? Could there be a role for data-center inspections to ensure hardware integrity, or might there exist opportunities for shared data centers in geopolitically neutral locations?3
II. Mechanisms are Vulnerable to Circumvention
The introduction of mechanisms for disabling or throttling on-chip components (via on-demand or other lock mechanisms) significantly broadens the potential for exploitation due to the expanded attack surface. Unlike methods where physical features are absent or where chips are not exported, for a mechanism that disables or throttles a feature, the regulated features need to be present on the hardware. In contrast to “hard-coded” mechanisms or inherent attributes, dynamic mechanisms inherently possess the required hardware features, making them susceptible to circumvention. For example, to limit chip-to-chip interconnect capabilities, one could simply reduce the available lanes and avoid installing high-throughput IO ports. In contrast, a chip designed to enable or disable features on demand must include all the physical components, regardless of their current use status.
Consider the “offline licensing” approach, where a chip contains its full computational potential, but access to this potential is regulated by licensing. If the licensing mechanism is bypassed, the chip’s entire power becomes accessible, negating the intended restrictions. Similarly, the “fixed set” approach allows for full-speed communication within a predefined set of chips but restricts it externally. If the mechanism enforcing this restriction is compromised, however, the communication limitations are rendered ineffective.4 I see the following primary threats:
Circumvention of Managed Features: The essential hardware features are integrated into the chip or board. If the on-demand feature is bypassed, the full capabilities become exploitable.
Compromise of Lock/Unlock Function: The functionality of flexible mechanisms requires a secure lock/unlock process, which suggests that an entity holds a private key for access. This entity, as the custodian of such a critical key, becomes a prime target for cyber-attacks, risking key leakage.5
Obsolescence of Restrictions: The choice of what the mechanism restricts becomes an ineffective proxy for risk mitigation. This could happen as a result of a new paradigm of AI that has a different computational profile.
For a comprehensive list of mechanisms that have been compromised, often those relying on on-demand features, see the Appendix PM me.
III. Prolonged Lead Times in Hardware Mechanism Rollouts
Implementing governance mechanisms through hardware necessitates the production and distribution of new chip generations, a process that takes time. For such mechanisms to become influential in the global compute distribution, they must be widely adopted and constitute a significant portion of the world’s computational power.
The effectiveness of hardware-based interventions is closely tied to the pace of technological advancement. Historically, the rapid pace of progress in computational performance has led to older generations becoming quickly obsolete. Should this pace decelerate, however, the desire to upgrade to new hardware diminishes, potentially undermining the governance mechanisms embedded within newer chips.
Assessing when to initiate the implementation of governance mechanisms — and the advocacy thereof — is essential for timely and effective intervention. This also helps to understand the impact of legacy chips (i.e., their total compute capacity retained through previous generations of hardware still in operation) which do not have the desired mechanisms and can therefore be used for potential evasion.6
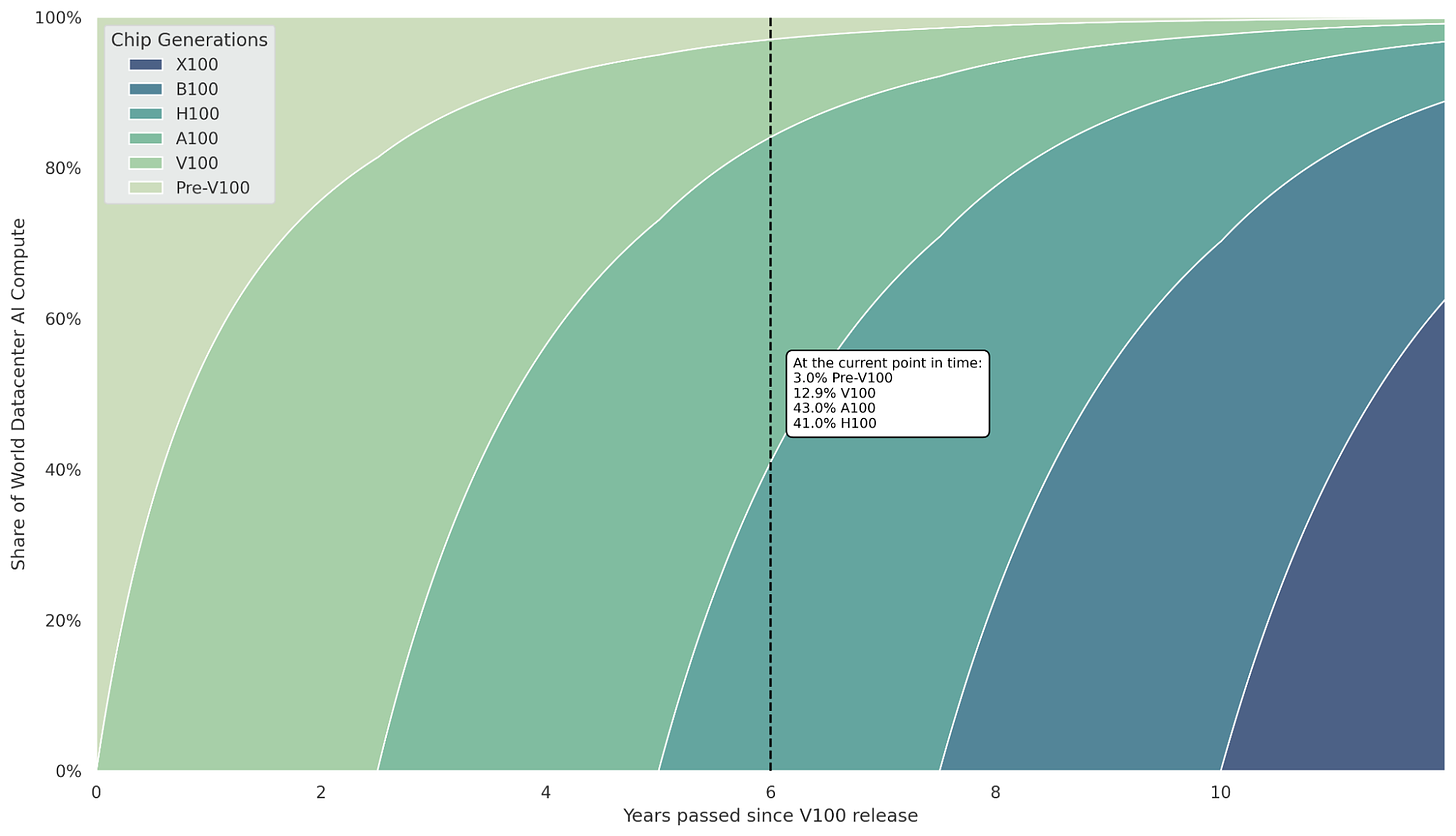
IV. Broader Considerations for Compute Governance
Beyond the arguments we explored in this blog post, which are special to hardware mechanisms, there is a wide range of crucial considerations and limitations of focusing on compute as a governance node. Despite the significant role compute plays in AI, many arguments contest its importance, suggesting that the governance capacity that compute enables can change. We differentiate between factors that impact the governability of compute itself and those that reduce the importance of compute for AI systems. See this blog post for a discussion of the considerations and limitations.
Compared to software/firmware vulnerabilities, the cost of compromising a device might scale with the number of devices. (A software vulnerability could allow one to basically scale “for free.”)
Determining what “good” AI functionality entails, and what falls under the umbrella of “bad” or undesirable, is not a trivial matter. Such definitions require a nuanced investigation of AI’s roles across different sectors, acknowledging its capacity as a general-purpose technology.
This discussion should extend to the various models of AI governance that exist or are proposed, from unilateral controls to collaborative international agreements and standards. Potential models include bilateral arms control agreements, joint development projects akin to a “CERN for AI,” or the establishment of an “IAEA for AI” to synchronize multilateral AI standards. Each of these governance models presents unique challenges and opportunities when considered in conjunction with hardware mechanism implementation.
For example, for the “fixed set” proposal, one could: (i) unlock the ability of higher bandwidth communication outside the pod, or (ii) decouple GPUs from the pod, potentially allowing for scaling within the pod or other pods.
Governance interventions could avoid this issue: a multilateral agreement could involve replacing unrestricted hardware and credibly demonstrating that the only remaining accessible compute implements appropriate controls.
Also - unrelated to anything in this article: Any plans to do a piece on the I-SOON leaks? I thought that was a great behind the scenes look at how Chinese cyberintelligence ops are farmed out to private companies.
Writing in from a software perspective - I didn't agree with a lot of the points raised about hardware based controls for restricting AI. IME hardware based controls around things like licensing end up being translated as FPGA code, which is well, code. Then you need controls around only allowing properly signed FPGA images, and preventing bypasses of that, it's turtles all the way down. You can make it harder to do training with large networks of chips, but people can just come up with new tricks to get around building large clusters, such as having different subdivisions that get combined at the end.
Zooming out a little, and tied to the discussion around safely storing cryptographic signing keys, the final product of AI training is a set of weights. These weights are huge (in terms of file size) and being the product are constantly being accessed. With crypto signing keys the best-in-class solution is to use special hardware (HSMs) to store the keys and perform limited operations on them that do not expose the key. These are hard enough for companies to use correctly, assuming the HSM is even fulfilling it's responsibilities of not leaking the key, and applying the equivalent hardware to AI weights just doesn't exist right now. Companies like OpenAI are heavily relying on their weights remaining private as their moat for consumers using them. In many ways I am reminded of the classic IRA quote on to Margaret Thatcher: "We only need to be lucky once. You need to be lucky every time" as applied to the problem of securing these high value assets. One leak is all it takes for those weights to be out there, forever.
The idea of private weights as a moat isn't scalable though. It's much more expensive to train models than to run them. Today places like HuggingFace contain large repos of open model weights, some of which can come quite close in performance to cutting edge private weights. These are further optimized to run on simpler platforms, such as someone's personal computer.
As the gap between public and private models narrows a better question in my mind is to ask what can be done to prepare for a world in which the average person has access to open weight models. The nuclear era (Restricted Data by Alex Wellerstein is a great read on this) focused on preventing the dissemination of knowledge (results could be "born secret" and not published) and making research onerous. Even then people managed to find better, cheaper, and easier ways to create nuclear devices.
This played out in the 90's already with cryptography as well. When the primary item being blocked is knowledge - people can find ways to transmit it. No amount of laws were able to prevent the spreading of cryptographic knowledge outside of the US and eventually laws restricting it had to be repealed because they were not effective and were holding back US innovation. I predict something very similar is going to play out with AI as well.